Introduction
Investment in emerging analytical technologies is growing fast, dominated by digital giants such as Google and Baidu, with companies spending more on R&D and deployment than ever before. Global revenue from the artificial intelligence (AI) software market alone is predicted to grow from $9.5 billion in 2018 to US $118 billion in 20251.
Against this backdrop, ambitious young enterprises in every industry are seizing opportunities on the strength of agile operating models, innovative ways of working, and the use of nimble solutions such as augmented analytics, continuous intelligence and graph analytics.
Industries from e-commerce to manufacturing are producing and analyzing data at a rate once unthinkable.
With the advent of the smartphone, smart spaces and internet of things (IoT), firms are quite literally drowning in data; in 2018, businesses produced over 1.5 quintillion bytes of data every day2.
Collecting, synthesizing and analyzing this data comprise a new science. The analytics function enables enterprises to become more intelligent, connected and insightful about what partners, employees and customers are thinking and doing. As data output increases, organizations can gain more accurate insights as algorithms are exposed to more training examples, learning, for example, to reject false positives and identify correct answers. In the manufacturing industry, predictive maintenance is a hot topic, brought about by new forms of data gathered from connected, smart equipment across the value chain. Managing downtime and predicting capacity are critical activities here; insight into these numbers can translate into millions of dollars saved and new revenues generated.
Many enterprises also understand that they need to adopt data management capabilities to stay ahead of the competition. Everything from data quality to master data management and data governance is crucial in this digital era. Firms must first focus on managing data that is most relevant to business outcomes, before putting it to work.
New technologies such as robotic process automation (RPA) and deep learning enable users to uncover what the data is saying, make it useful, and ensure that it’s available to support enterprise decision-making at the point of insight. These technologies enable companies to anticipate demand, improve sourcing, optimize R&D and hence produce higher-quality goods at lower cost. Analytical prowess and robotic intelligence, including virtual agents, allow customer-facing firms to provide personal, rich and convenient experiences for both users and clients.
“The ability to both understand and predict circumstances allows us to move forward proactively,” said one leader from the automotive industry. “We are then able to enhance our own processes internally and also reach out proactively to customers, often before they may even realize there’s an issue. We can be the ones to alert them about the situation and let them know how we’re already fixing it for them. It takes a lot of coordination and collaboration around data within the organization.”
So how are firms using these insights to transform their business and operating model? What is their appetite for automation and AI? What are the biggest values to be realized from retooling the enterprise to become an AI thinker? If partnering is the way forward, what is the viability for complementing this expertise with in-house data science and commercial off-the-shelf solutions such as RPA and deep learning? Is it more important for a partner to have expertise in emerging tech, or is the ability to instigate cultural change more favored by incumbent organizations?
Infosys is a leading digital consulting and services firm. In March 2019, it commissioned independent research that surveyed 154 senior leaders from seven industries, including financial services, high tech and manufacturing. Respondents hailed from Australia, Canada, Europe, New Zealand and the United States.
The key findings are presented in this report.
Executive summary
- Operational excellence and cost efficiencies, rather than new opportunities, are the two biggest values to be realized from retooling the enterprise from a rule-based enterprise to an AI thinker.
- Eighty-one percent of the respondents believe that insight is important and aids in automating core systems or processes by applying cognitive and machine learning (ML) technologies.
- Respondents cited high levels of investment in cloud (44%), automation (35%), IoT (29%), and AI or ML (29%) to drive advanced analytics and big data efforts.
- Citizen analysts – non-data scientists who can do sophisticated data analysis – are in short supply. Only 13% say their employees have the skills, with a further 49% saying they have the tools but not the expertise.
- Seventy-two percent say that AI and ML are used to some extent to automate complex processes that currently require human judgment. Automation of more complex processes to some extent is most prevalent in manufacturing (87%), telecom (80%) and high tech (78%).
- Only 35% of organizations use the exhaust – the trail of data left by activities of internet users from various systems – to discover new opportunities, innovate and improve the business.
- For generating insights, the preferred approach is to use a combination of in-house efforts (developing data science and data governance); readymade tools, solutions and platforms; and external partners (31%). In total, 68% use external partners in some way.
- When generating insights, enterprises expect their IT partners to help across the full spectrum of people, processes and technology. Assistance in driving cultural change, innovating the business and developing capabilities around emerging technologies factored high for partner preference.
Evolving to a data-driven enterprise
In any digital transformation initiative, a modernized core is foundational: Once armed with a modern, scalable and flexible core, the enterprise can build the analytical capabilities it needs around that digital footprint, accelerating the move toward customer, partner and employee centricity. As an organization matures in this core transformation, it becomes more confident and ready to face disruption head-on. New challenges arise as more initiatives are scaled out, but addressing them becomes easier as the workforce matures and agile ways of working are instilled.
Additionally, as the enterprise becomes more data-driven, new thought leadership within the enterprise accelerates growth. This knowledge, built internally through data science, design thinking and innovative practices, or externally with partners, fosters new use cases to deliver products and implement processes faster, better and more cheaply.
A recent Infosys report3 found that visionary organizations – those that will ultimately win the war of digital disruption – quickly appropriate the opportunities that cognitive technologies offer. They “think, move and act like digital natives”, transforming both culture and business model in the process. Instead of merely cutting costs and thinking about the bottom line, these incumbents bring innovative ideas and emerging technologies to scale. They keep in step with customer aspirations and market forces, including the threat of new entrants and obsolete operating models.
However, most firms surveyed for this report haven’t taken this bold step yet; more often than not, they explore different avenues that impact the bottom line and win immediate return on investment (ROI).
When asked the value of retooling the enterprise to become an AI thinker, 45% of leaders were interested mainly in “keeping the lights on,” naming “operational excellence” as the main driver of transformation (Figure 1).
Cost efficiencies received 19% of the vote. Only a tenth expect to realize value from innovative ideas and technologies. As the vice president of a New Zealand-based large utilities firm said, “With AI, automation and analytics, we are looking to automate processes in order to be more efficient.”
The transformation journey needn’t be just about cutting costs and improving efficiency. With the right systems and processes in place and a focus on generating talent within the enterprise, better customer experience, innovative ideas and a paradigm shift in the business and operating model will emerge, preparing the enterprise for the future of work.
For this to occur, a solid road map must first be outlined. This strategy must have support from business leaders, and must amplify core parts of the business while enabling partners to take the lead on forefront digital initiatives.
Figure 1. Firms are more interested in operational excellence and cutting costs than innovating, as they become AI thinkers
Value realized from re-tooling the enterprise to become an AI-thinker
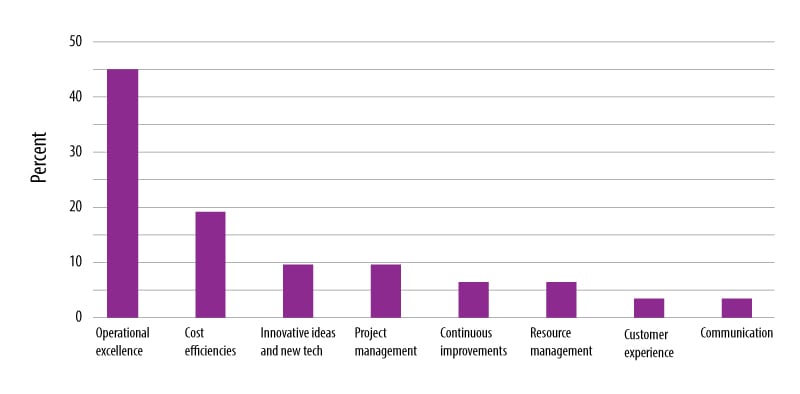
Source: Infosys Knowledge Institute, 2019
Cloud, automation, IoT and AI – top investments to drive analytics
With data, and the technologies that use it intelligently, any small advancement is a ripple that permeates outward, rapidly influencing the whole enterprise.
In this research, 81% of the firms reported they believe it’s at least somewhat important to glean insights by applying cognitive technologies and analytics (Figure 2).
Studies and industry practitioners who tout AI, advanced analytics and big data as major trends across sectors back this finding.
Augmented analytics, a third major wave for analytics capabilities, is one of Gartner’s top strategic trends for 20194.
In the AI space, Amazon is proactively working on speech recognition and robotics, BMW and Tesla are committed to delivering driverless cars in the next five years, and IBM has invested US $3 billion in IoT, making its Watson cognitive computing service a force in the sector.
In this research, senior leaders name cloud (44%), automation (35%), IoT (29%), and AI or ML (also 29%) as the top investments to drive analytics and big data efforts (Figure 3). For cloud, the numbers are even higher in the IT space, with 62% of CIOs rating cloud as a high investment. DevOps is further down the list, with 26% of the vote, though companies in Australia and New Zealand see the solution as more critical to digital success (both scoring 37%) than their counterparts in the United States.
Figure 2. 81% of the firms say that gleaning insights by applying cognitive technologies is important
How important is it for an organization to glean insights by applying cognitive technologies?
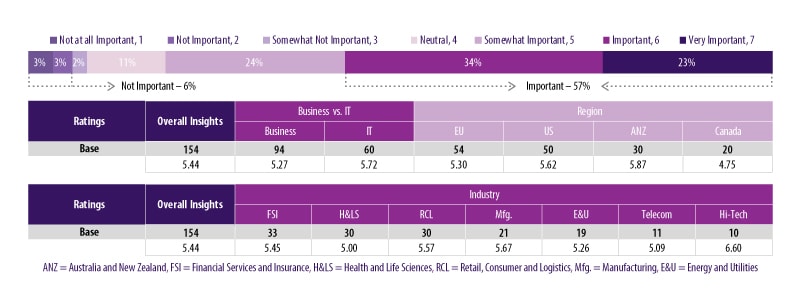
ANZ = Australia and New Zealand, FSI = Financial Services and Insurance, H&LS = Health and Life Sciences, RCL = Retail, Consumer and Logistics, Mfg. = Manufacturing, E&U = Energy and Utilities
Source: Infosys Knowledge Institute, 2019
Figure 3. To drive advanced analytics and big data efforts, cloud, automation and AI get the lion’s share of investment
What is the level of investment in the following technologies to drive advanced analytics and big data efforts?
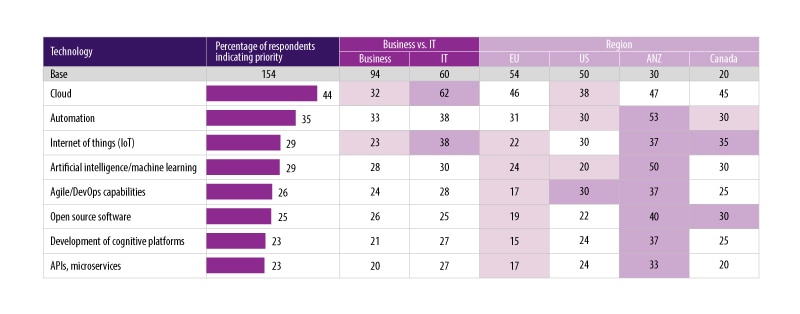
In the report “Core Modernization as a Catalyst”5, cloud also received the highest priority for core modernization investment, with a similar 44% of the respondents naming it as their top modernization initiative. This turn to the cloud will become more prescient as firms are now inundated with data from sensors, social media and unstructured data sets generated by AI. The cloud offers more powerful processing capabilities so that AI can train and execute algorithms at scale.
Donald Feinberg, vice president at Gartner, says, “The size, complexity, distributed nature of data, speed of action and the continuous intelligence required by digital business means that rigid and centralized architectures and tools break down. The continued survival of any business will depend upon an agile, data-centric cloud architecture that responds to the constant rate of change.”
Some cloud analytics packages, like Adobe, Oracle and Salesforce, are a good business solution for enterprises looking to bolster their digital marketing and customer-facing capabilities. With processing carried out in the cloud, ML and advanced statistical models dig automatically through business data, searching for patterns that data scientists often don’t have time to analyze. This capability also gives rise to a new model of citizen analysts, a business environment where many different people within an enterprise can collaborate on data analytics and share ideas that work well.
“This process of letting people collaborate on data freely, with checks and balances from hard-core analysts, creates a system where data’s value and context become clear,” says Mohanbir Sawhney, marketing professor and co-author of The Sentient Enterprise: The Evolution of Business Decision Making6.
In our research, these citizen analysts are in short supply. Only 13% of organizations say their employees have the necessary data analysis skills and are actively working with data scientists in the enterprise, with their numbers highest in telecom (20%) and manufacturing (20%). A further 49% say they have the tools but not the expertise, and 24% say that they rely only on qualified data scientists (Figure 4).
According to Gartner, through 2020, the number of citizen analysts will grow five times faster than the number of professional data scientists7.
As the open economy and sharing of data throughout the partner ecosystem gathers steam, these analysts will use AI-powered augmented analytics tools that automate the data science function, identify patterns in data and develop new hypotheses that may be missed by more experienced professionals.
Our data, however, reveals that incumbent firms have a good way to go before information sharing and data analytics are scaled up across business processes, with employees acting as levers for more effective business strategy. As the authors of The Sentient Enterprise: The Evolution of Business Decision Making point out, a good system for analytics might recommend other people in the company working on similar projects, just like Facebook and LinkedIn might recommend friends, or Amazon might recommend products based on user history. This sort of platform then creates a community within the enterprise, and employees can ask advice around analytics and can like, share and up-vote the most effective insights.
Figure 4. Citizen analysts are in short supply, with most respondents saying they have the tools but not the necessary experience
What is your organization’s position on using citizen analysts?
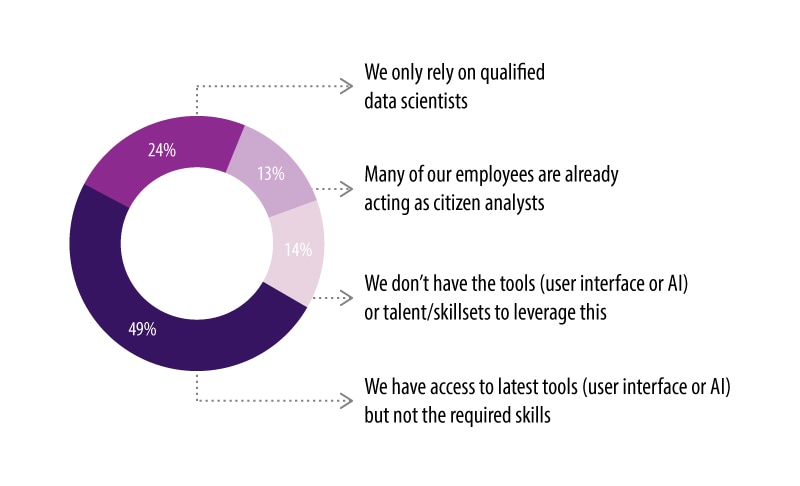
Source: Infosys Knowledge Institute, 2019
Using AI and ML to automate complex processes
Using cognitive technologies or ML to automate core systems and processes is crucial if incumbent firms are to keep up with digital natives.
Every 11.7 seconds, Amazon deploys code to production. Netflix employs thousands of software updates every day using DevOps. On the other hand, those without a high dose of cognitive technology in their DNA struggle to release software even once a month8.
In the banking sector, there are clear benefits from improved speed and accuracy in AI-optimized fraud-detection processes, which is forecast to be a $3 billion market in 20209.
Performance in certain industries, including high tech, manufacturing and telecom, is driven by automated forecasting, fast and accurate processes, and personalized customer connections at the user end of the supply chain. The Netflix algorithm that personalizes recommendations to over 130 million subscribers worldwide is a critical part of the company’s success. Through better search, Netflix estimates that it saves US $1 billion every year in lost revenue from users who might otherwise cancel subscriptions due to flawed video recommendations10.
Additionally, rapidly changing expectations around data mean that with these new technologies woven into front- and back-office processes, firms can also match customer expectations of “real-time interaction.” Customers now demand low latency. Google found that it takes only 250 milliseconds for a user to get upset with a faulty website11. Such stories are driving a new self-service ethic where users, and increasingly employees and other partners, expect to access data rapidly and in ways that suit their needs. Automation and particularly intelligent algorithms driven by deep learning are the way forward.
In this research, automation of more complex processes (to some extent) is most prevalent in manufacturing (87%), telecom (80%) and high tech (78%), with financial services further behind (69%). In retail, 73% automate complex processes to some extent, while the health industry is one of the laggards in this context, with 65% of the vote. Use cases in retail are numerous, though there are compelling benefits from automated customer operations, improved inventory forecasts and targeted promotional campaigns, segmenting customers based on a whole catalog of attributes. In health care, AI-powered treatment systems can deliver optimized outcomes for patients, save costs and deliver substantial ROI.
Figure 5. Most firms automate complex processes to some extent, with a fifth automating simple processes to a large extent
To what extent does your organization use AI/ML for the following processes?
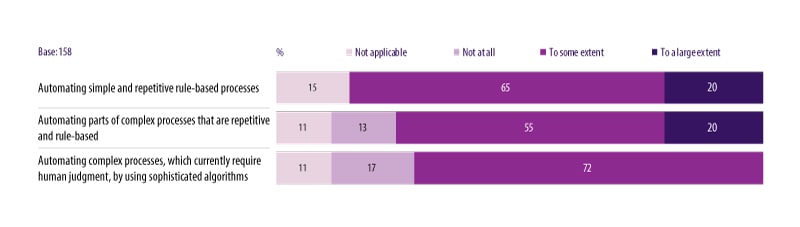
Source: Infosys Knowledge Institute, 2019
Infosys assisted a leading global bank in transforming its operating model with automation
Infosys assisted a global bank in introducing a new operating model to its South American operations, creating small teams of cross-functional experts working together in sprints.
The bank implemented a tooling framework featuring agile DevOps teams to ensure continuous integration of the code. Automated quality checks were then carried out. Sophisticated test management tools performed unit, system and nonfunctional testing. To increase release frequency, the technical solution was deployed automatically to production. Rigor and speed were integrated into the DevOps process based on principles such as “no manual deployments” and “minimum operating standards” for all code released into production.
The result: significant cost optimization through direct savings and absorbing headwinds, a sizable improvement in IT quality and service stability, and greater than 10% improvement in key performance indicators.
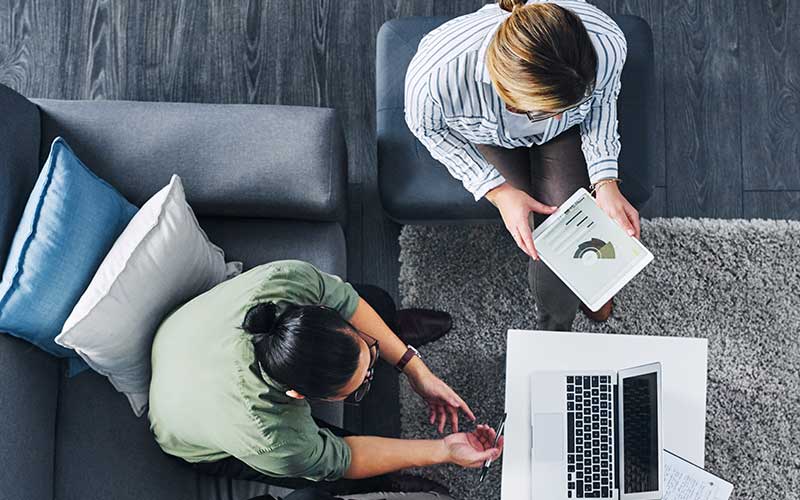
Challenges of building a customer-centric business model
Another finding from our research is that organizations generally use “data exhausts” to improve system performance and “keep the lights on” (Figure 6). Only 35% use the exhaust to discover new opportunities for innovating the customer experience and improving the business, though the numbers in manufacturing (47%), high tech (44%) and telecom (40%) are higher on this metric.
This tallies with the findings mentioned earlier that operational excellence and cost cutting are the main drivers behind retooling the enterprise to become a data-driven AI thinker.
When used correctly, however, data intelligence gives rise to a self-service culture, wherein both customers and employees solve new problems at the point of insight (known as “proximity”). Within the firm, silos are broken down as this intelligence is made more transparent (through technologies such as virtual data marts), and new perspectives, both internal and external, are born. Knowledge is shared faster through the enterprise, including through predictive analytics targeting customers and employees. These insights – the “lifeblood” of the enterprise – then inform a host of important decisions: for example, how to find new opportunities, uncover hidden threats, discover new needs, identify unknown problems, create better experiences, save resources and amplify talent.
Amplifying talent in house can expedite the move to customer-centric business and operating models while mitigating the AI talent shortage around the world.
According to a report from Indeed.com, job postings for “machine learning engineer” had the highest three-year growth rate of any job, increasing more than four times from 2015 to 201812. In response, firms are adding people to their AI and data science teams with broader skill sets, such as knowledge of linguistics and politics, and training them. Of course, it’s important to have AI and data analytics “rock stars,” but not every person needs to be one. When enterprises build a more diverse knowledge economy (which they can also do by finding the right partners), surprising results emerge, such as when a data-science employee without a traditional analytics background adds depth and color to an analytics problem.
Figure 6. Data exhaust is used primarily to improve system performance, rather than innovate the business
What is your organization’s position on using citizen analysts?
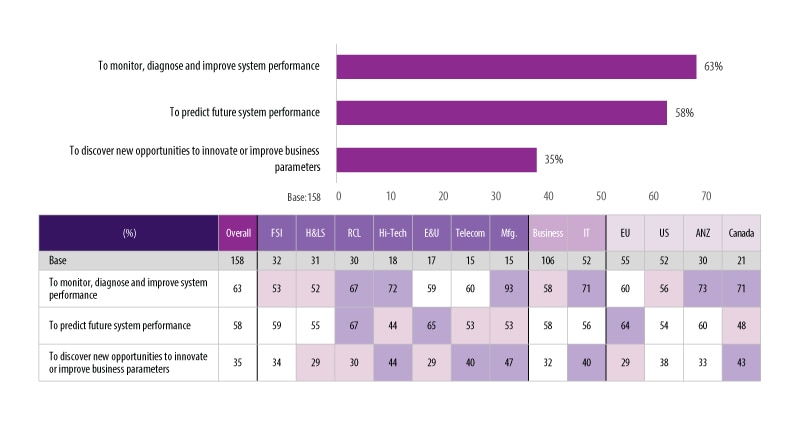
Source: Infosys Knowledge Institute, 2019
How Infosys transformed insights into forecasts for a retail client
Reverse-engineering historical data to arrive at the present reality was easy for one large retailer. More difficult was the ability to identify patterns that would repeat in the future, enabling the retailer to automate demand forecast accurately.
Parameters for this forecast included both historical and evolving sales, and channel and market ecosystem data. Infosys introduced an automated demand-forecasting platform that harvested demand intelligence in real-time. Using the most suitable statistical method and time horizon, the new platform gave the retailer a holistic view of channel-wise traffic and demand with minimal forecast error.
The impact of this new platform was powerful. The retailer was able to predict sales by channel and product category with an 85% success rate. The retailer now has better visibility into short-term order volumes and best-selling products, along with higher revenue growth and improved seasonal planning.
Build analytics and automation in house or by partnering?
Big data and the new analytics capabilities that accompany it change the nature of the enterprise: everything from the way it is structured and finances operations to how it engages the workforce and pursues opportunities. Organizations make decisions in near real-time as they listen to data sources and use advanced analytics, including natural language processing, to understand what the data is saying. So how can firms actually procure and use advanced analytics tools to deliver insights, strengthen the core of their business model and provide value to customers?
In the report “Customer experience: An Imperative for Digital Transformation”13, research found that seven out of 10 respondents were keen to partner with external solution providers to build customer journeys. Only 10% of business and IT leaders were developing everything in house.
For generating insights using advanced analytics and big data, the preferred approach is to use a combination of in-house efforts, ready-made tools and solutions (RPA, ML and deep learning products and platforms), and external partners (31%). In total, 68% use external partners in some way. Pure in-house efforts around developing data governance, data democratization and data science capabilities received 14% of the vote (Figure 7).
When partnering, however, enterprises in our research expect these relationships to boost capabilities across the full spectrum of people, processes and technology (Figure 8).
Driving the required cultural change to deliver insight across the enterprise was the highest-ranked capability looked for in a partner, scoring 5.32 on a scale of 1 (not at all critical) to 7 (very critical).
Assistance in innovating the business and providing thought leadership (5.3) as well as capabilities around emerging technologies (5.3) also factored highly for CXOs.
As the CXO from a large manufacturing firm in Europe said, “To survive in the era of new digital competition, an organization must begin its transition to an intelligent enterprise with the culture before moving on to the technology.”
Finding the right partner is a decision that should be made carefully. By sharing internal data with a partner fully, at scale, cyber risk increases; the partner’s data governance and business process policies must also be taken into account. A company must ask the question “Does this partner provide access to complementary skills and resources that will amplify existing projects, while leaving us room to work on more long-term assignments?”
In general, partnering increases chances of success and benefits from much quicker implementation (Figure 9).
For firms further along on their data-driven transformation, effective partnering is easier to achieve, as processes and governance within the enterprise are more mature.
Figure 7. For generating insights, firms prefer a combination of developing efforts in house, partnering, and adopting sophisticated AI technologies and platforms
What is your organization’s approach to generating insights using advanced analytics and big data?
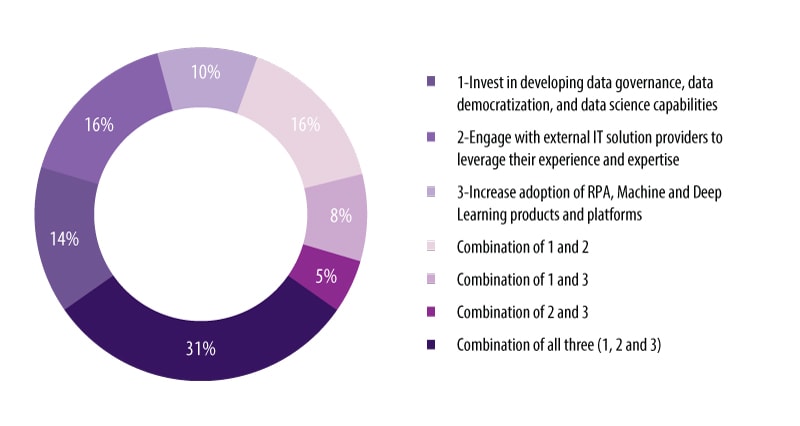
Source: Infosys Knowledge Institute, 2019
Figure 8. Insight partners should drive the expected cultural change within the organization, along with innovation and thought leadership capabilities
How critical are these capabilities in an external solution provider to deliver insights across an enterprise?
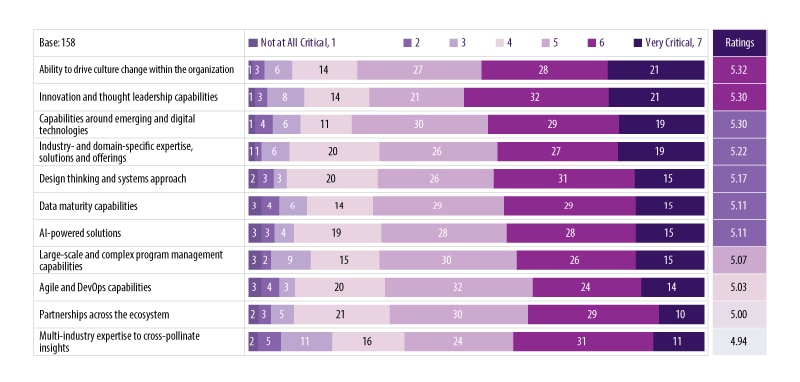
Source: Infosys Knowledge Institute, 2019
Figure 9. Higher chances of success and quicker implementation are the top two considerations for partnering
Why do firms partner?
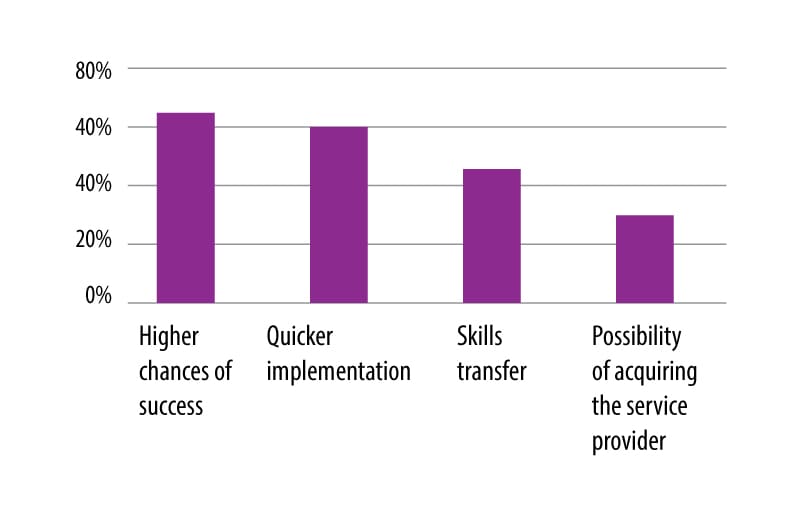
Source: Infosys Knowledge Institute, 2019
How Infosys transformed insights into forecasts for a retail client
Organizers of the French Open 2019 believed that a new raft of digital innovations, including the use of analytics, augmented reality and virtual reality, could elevate the importance of the tournament in the public consciousness.
Infosys helped event organizers deliver the tournament’s digital transformation objectives – enabling it to remain at the cutting edge of technology and to provide tennis fans with an even richer experience.
A new easy-to-use tool for the official Roland Garros website delivered live insights and scores generated from data analytics, augmented reality and virtual reality applications. This tool allowed fans to experience what it’s like to play a game on Court Philippe-Chatrier. Players and coaches also had access to AI-powered data and video analytics throughout the tournament, helping players improve serves and movement around the court.
Conclusion
Higher ROI, new revenue streams and significant competitive advantage will go to those firms that use data analytics and cognitive technologies as part of their business strategy. In fact, enterprises that focus on data-driven decision making have an average of four percentage points higher productivity and six percentage points higher profits than those that don’t 14.
In this research, most are using analytics and adjacent technologies mainly to improve efficiencies and “keep the lights on”. Instead, firms must concentrate on quick-win use cases where insights lead to new capabilities that fundamentally change the way the business makes money. Operating the business can then be based on data-driven decision making, and predicting the next big thing will be based on facts rather than hunches. Confidence in one initiative - automation of back-end processes, predicting supply chain performance, optimizing the customer journey, among others - will then light the touch paper for deploying more use cases at scale.
Some digitally-advanced enterprises in this research are investing at scale in related analytical technologies such as business intelligence and cloud computing.
These firms, often found in manufacturing, telecom and high tech industries, are advanced enough to address multiple use cases in parallel. They are more likely to adopt direct-to-consumer models and use artificial intelligence to automate complex processes that once required human judgement.
To follow suit, digital laggards must make careful yet definite changes across all people, processes and technologies. A trusted partner that can change the working culture of the enterprise is important here, as is the ability to cultivate thought leadership and procure technologies in step with business strategy. Firms should also partner to develop talent and build up infrastructure as early on as possible. Only then will they catch up with the adoption curves of these advanced corporates.
The ultimate goal is to create a business environment where many different people within the enterprise can collaborate on data analytics and share ideas that work well. Here, a system is constructed where data’s value and context becomes clear. The result? Business-wide change across the value chain and a corporate culture that frees employees and partners to concentrate on more interesting and productive work.
References
- Revenues from the AI software market worldwide from 2018 to 2025, Statista
- Data never sleeps, Domo
- Digital Radar 2019: Enabling Transformation through Salesforce
- Gartner Top 10 Strategic Technology Trends for 2019, Gartner
- Core Modernization as a Catalyst, IKI
- The Sentient Enterprise: The Evolution of Business Decision Making, Oliver Ratzesberger and Mohanbir Sawhney, Wiley
- Gartner Top 10 Strategic Technology Trends for 2019, Gartner
- 10 companies killing it at DevOps, TechBeacon
- McKinsey Global Institute – Artificial Intelligence: The Next Digital Frontier
- Nathan McAlone, “Why Netflix thinks its personalized recommendation engine is worth $1 billion per year,” Business Insider, June 14, 2016
- “For Impatient Web Users, an Eye Blink Is Just Too Long to Wait,” The New York Times
- Businesses Build AI, Data Science Teams with Nontech Talent, WSJ Pro
- Customer experience: An Imperative for Digital Transformation, IKI
- What Makes a Company Good at IT?, Wall Street Journal, 2011
Survey methodology
In March 2019, Infosys commissioned independent research, attracting responses from 154 senior leaders from seven industries, including financial services, high tech and manufacturing. Respondents hailed from Australia, Canada, Europe, New Zealand and the United States.
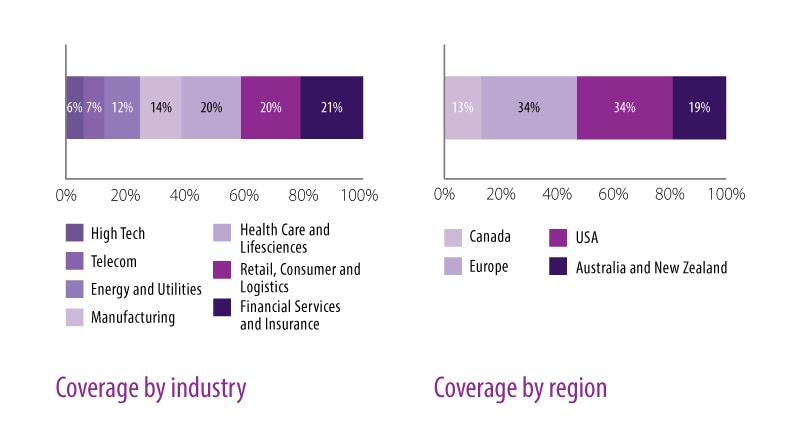