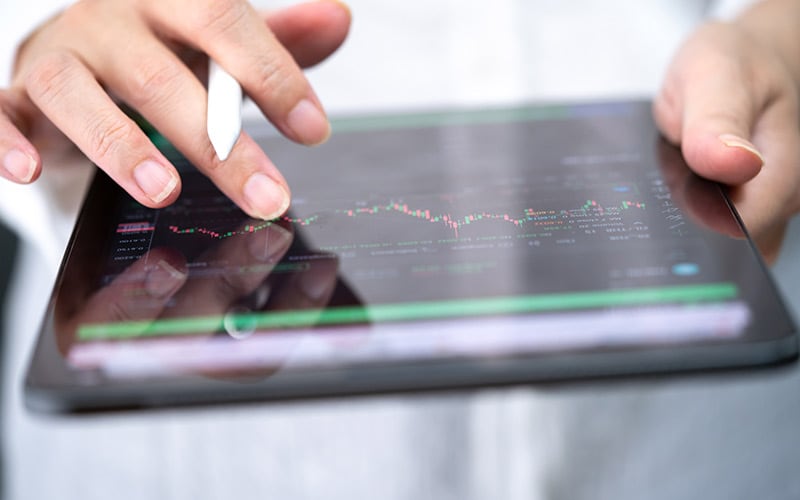
Insights
- The financial security issuance process can last from a few days to several months and involves multiple stakeholders — issuers seeking capital, regulators ensuring market integrity, underwriters managing risk and distribution, and investors pursuing returns.
- Yet the issuance process is fraught with challenges including:
- Stakeholder coordination
- Lack of standardized documentation
- Unstructured and complex data
- Scalability
- Need for specialized talent
- Manual processing
- Al-powered automation that combines traditional and generative AI can improve the underwriting processes.
- Tenets of an AI-powered platform include:
- Integrating AI models
- Al governance
- Human-in-the-loop approach

How Palo Alto Networks and Infosys are leading with AI in cybersecurity
Discover how Infosys and Palo Alto are helping enterprises reduce risk, improve response times, and consolidate tools through platformization.
Financial securities are the lifeblood of the financial world — tradable instruments that embody ownership (stocks), debt (bonds), or rights to ownership (derivatives) — and are pivotal for corporations, governments, or financial institutions to raise capital from investors for expansion, restructuring, funding infrastructure projects, or boosting liquidity.
The issuance process includes identifying funding needs, structuring the security, obtaining regulatory approvals, working with underwriters and other intermediaries to price the securities, and distributing the securities to investors. Depending on the type of security and jurisdictional requirements, the process can last from a few days to several months.
This financial ecosystem engages diverse stakeholders: Issuers seeking capital, regulators ensuring market integrity, underwriters managing risk and distribution, and investors pursuing returns. Post-issuance, these interactions focus on compliance monitoring, performance evaluation, and facilitating secondary market trading.
In 2023, global fixed income and equity market capitalization surpassed $140 trillion and $115 trillion, respectively. These figures are a powerful catalyst driving opportunities for innovation throughout the financial security ecosystem.
Challenges in capital market operations
As securities issuances are vital to the economy, managing market dynamics presents a significant challenge. Market participants face several issues, including:
- Stakeholder coordination: Multiple institutions involved in securities issuance must work together smoothly. Weak coordination can lead to miscommunication, added complexity, and increased risks.
- Lack of standardized documentation: Nonstandardized documents across institutions complicate drafting, reviewing, and managing securities paperwork. This slows the process of securities issuance, creates inefficiencies, and raises the risk of noncompliance.
- Unstructured and complex data: Securities documents often contain large amounts of text, tables, and footnotes that are difficult to analyze. This unstructured data leads to inefficiencies and processing delays.
- Scalability: New types of securities require detailed and customized documentation to comply with operational and regulatory demands. This adds complexity, increases workload, requires more experts, and faces stricter oversight.
- Need for specialized talent: Financial institutions need experts who understand securities issuance and regulations. Hiring and retaining these professionals is expensive due to their limited availability and high demand.
- Manual processing: Tasks before and after closing are repetitive, labor-intensive, and prone to errors. These processes rely on verification systems that increase costs, delays, and the chance of mistakes.
Effective solution: Al-powered automation built on an AI-driven architecture
The existing underwriting process runs on legacy systems that lack automation and have fragmented workflows. It involves manual review of extensive — often unstructured — documents and subsequent data entry. Due to the high volume of manual operations and checkpoints, it is time-intensive, costly, prone to human error, and requires substantial business expertise across all asset classes.
Traditional AI and machine learning (ML) models have been used extensively — the financial services industry invested $35 billion in AI in 2023. The technology helps institutions automate data digitization and extraction platforms, boosting efficiency in processing structured and standardized documents such as invoices and financial statements. It also helps streamline transaction processing, portfolio management, and audits.
However, another significant challenge is that securities issuance documents are unstructured, making the process difficult to automate. This challenge is exacerbated in capital markets and underwriting, where document structures vary across institutions and securities, further complicating standardization and automation.
Figure 1. An AI-powered platform built on an AI-driven architecture
Note: UI – User interface
Source: Infosys
An AI-driven architecture combines traditional and generative AI to improve underwriting processes. The main benefits include:
- Less manual work: AI automates data extraction and review, reducing errors and costs while speeding up workflows.
- Faster turnaround: AI processes data much quicker than manual systems, enhancing productivity and responsiveness.
- Unified platform for multiple assets: AI handles multiple financial instruments in one workflow, simplifying operations.
- Centralized documentation: AI extracts and organizes data from various documents, improving audits.
- Adaptability to new assets: AI can easily adapt to new assets and regulations, ensuring smooth operations.
- Consistent processes: AI applies uniform rules for accurate and transparent data processing.
- Efficient operations: AI automates routine tasks, allowing smaller teams to focus on complex tasks.
In our work with clients, this architecture has:
- Improved the average data extraction cycle time by 90%.
- Reduced human errors by at least 80%.
- Improved onboarding of new/complex instruments by 90%.
- Reduced document processing cycle time from around 80 hours to just one hour.
Importantly, this architecture reduces risks and frees up expert resources to focus on high-value tasks. Its features enable scalability and adaptability, creating a competitive advantage for capital market institutions.
Tenets of an AI-powered platform
As each institution’s needs are unique, the first step is prioritizing specific use cases. Then, a minimum viable product is developed to test the solution’s effectiveness, helping businesses address key concerns and gain immediate value from the platform implementation.
Integration and scalability into the platform's initial design are essential for institutions, ensuring the platform accommodates future growth and adopts new requirements. A scalable design facilitates seamless modifications and enhancements, helping enterprises meet dynamic demands efficiently.
1. Integrating AI models
AI and ML platforms streamline securities document processing by classifying, validating, and extracting data. Since documents contain data in various formats like text, tables, and titles, these platforms use an ensemble of models — such as BERT-CNN, RoBERTA, QnA, and large language models (LLMs). Using these models makes platforms adaptable to different document types and asset classes, enabling fast and accurate data processing regardless of format or location. AI models turn manual document handling into an automated, user-friendly, and cost-effective platform (Figure 2).
Figure 2. Workflow in an AI-powered platform
Note: OCR – Optical character recognition ; NLP – Natural language processing
Source: Infosys
Institutions leveraging advanced technology platforms with AI and ML models can deploy these solutions within their infrastructure, depending on model size, bandwidth, and computational needs. With the growing adoption of LLMs across technology companies, organizations can invest in dedicated graphics processing units (GPUs) for their infrastructure or use cloud-based generative AI platforms. PayPal, for example, used accelerated computing by upgrading its AI infrastructure with GPU utilization to reduce its cloud costs by 70% and runtime by 35%.
2. Al governance
Robust governance is crucial for the responsible use of AI in document digitization and processing. Effective data governance protects data integrity, privacy, and security, especially in AI systems handling large volumes of sensitive information. These principles help minimize errors and safeguard sensitive data. Compliance with laws and regulations is also key, particularly in finance, where human oversight, regular audits, and ongoing monitoring are necessary to ensure fairness, accuracy, and compliance with standards.
AI governance includes monitoring and auditing to ensure models work correctly and produce accurate results. It promotes accountability and allows for algorithm fine-tuning. Effective governance reduces risks, encourages teamwork, and provides reliable insights for decision-making. Additionally, roles can be assigned based on authority levels, such as allowing one user to view results, another to audit, and a third to make changes.
3. Human-in-the-loop approach
Human oversight is essential to ensure fairness, accuracy, and compliance with standards. Effective AI governance includes human oversight and promotes responsible and ethical decision-making. Subject-matter experts (SMEs) play a key role in curating data for AI model training, ensuring it is relevant and aligned with the desired outcomes. The human-in-the-loop approach allows SMEs to actively participate in developing and validating algorithms for accuracy and reliability. Human involvement also extends to regular auditing of AI systems, especially to assess performance in handling exceptions. This oversight ensures AI-driven decisions are fair, accurate, and meet legal and regulatory standards.
Benefits of an AI-driven approach to issuance
The tenets and benefits of an AI-driven architecture can be implemented by various stakeholders in the securities issuance process:
- Asset managers can use Al-powered platforms to extract key data points, enabling deeper insights into investment vehicles, their limitations, and associated risks.
- Trustees and paying agents utilize these solutions to calculate and disburse interest payments accurately while simplifying compliance and reporting workflows.
- Law firms can use the platforms to update documents accurately or reduce review times.
- Rating agencies can integrate the extracted data and output of these platforms into proprietary cash flow models or use them to prepare detailed analytics for committees.
- Financial infrastructure companies may utilize Al-powered platforms within their underwriting or corporate actions departments to enable more efficient processes for key data extraction.
The integration of AI-powered platforms into the securities issuance process has brought about a transformation into a traditionally cumbersome sector. With advanced AI models alongside robust governance frameworks, capital market institutions can streamline document processing, ensure rigorous compliance, and bolster decision-making capabilities. Human oversight in traditional and generative AI systems serves as a safeguard, ensuring that AI-driven outcomes remain accurate and unbiased. The future of securities issuance is inextricably linked to AI advancements, preparing the industry to become agile, responsive, and better equipped to navigate the complexities of modern finance.
