Knowledge Institute Podcasts
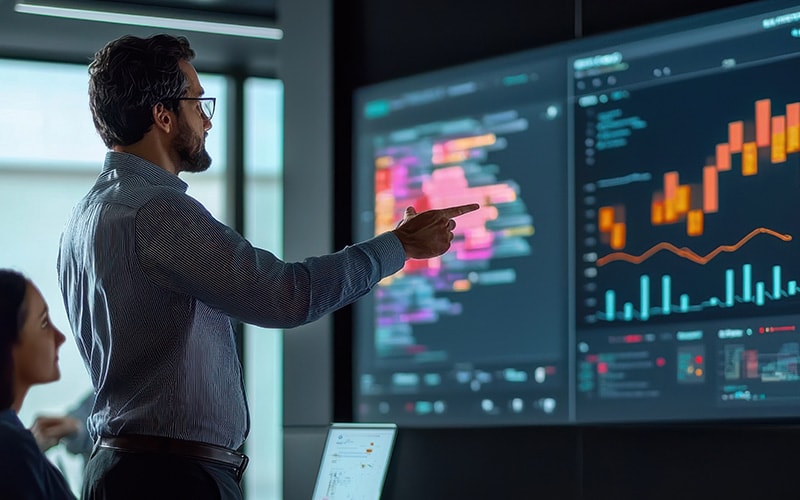
-
Data Democratization with Databricks: AI, Open Source & Business Transformation
February 28, 2025
Insights
- Companies that treat data as a strategic asset rather than an operational byproduct make faster, more informed decisions. A well-structured data ecosystem enables seamless AI integration, driving innovation and agility.
- AI is only as good as the data it learns from, making governance and structure essential for reliable insights. Without high-quality data, even the most advanced AI models risk producing misleading or biased outcomes.
"By listening to this podcast, you hereby acknowledge and understand that your personal data may be processed in the manner described in our Privacy Statement"

Chad Watt: Welcome to Ahead in the Cloud, where business leaders share what they've learned on their cloud journey. I'm Chad Watt, Infosys Knowledge Institute, researcher and writer. Today I'm speaking with Dael Williamson, the EMEA CTO of Databricks, and Rajeev Nayar, VP and CTO of Data Analytics for Infosys. We're talking cloud, data, AI and all things digital today. Welcome to you both.

Rajeev Nayar: Thank you.

Dael Williamson: Thank you.

Chad Watt: Dael, tell us a bit about Databricks and your role as EMEA CTO.

Dael Williamson: So Databricks, we are the data and AI company. And we have a purpose of enabling every enterprise to combine all of their data, definition broad, files, structure data, images, you name it, and then to democratize access to data and AI. So my role as EMEA CTO, I work with our biggest customers, all types, cross enterprise to commercial, digital, AI natives. I sponsor large field of initiatives where we are trying to figure out how to help customers on their journey.

Chad Watt: Before you were working in data cloud and AI, you were a biochemist. What led you to Databricks?

Dael Williamson: So I get asked this a lot. So I worked in a very applied setting. I worked in drug discovery. I worked in environmental waste bioremediation. And what I would do is I would leverage real world data, I'd generate synthetic data, and the focus was very much on proteomics. Back then it took forever to do anything, 2007 for example, like 45 to 185 days to run a simulation. With the tools that we have today, that type of workload, which was pretty advanced, but still rudimentary to what you're trying to solve, you're looking at hours to days. So you're taking something that used to take about 185 days and you're kind of bringing it down to maybe two days, and that's in two decades. So it's an insane amount of change. But if you think about what I used to do, I work with the same tools, I just work in a more generalized setting.

Chad Watt: Enterprises have a lot of data, and are spending time and energy to gain insights, and make more use out of that data. How is that going?

Dael Williamson: Not great. I'd say about the bulk of enterprises, a good high percentage, I'm not going to try and actually jump as to what, but it would be upward of 85% plus, they are really struggling to get their data house in order. Their data is everywhere. The older the company, the bigger the company, the more they have this kind of inherent proliferation. And they've set to work for many years trying to get it in the right place, that's driven through regulation, some of that's driven through risk. Some have done it, but they're sort of more the exception than the rule, and most of them are kind of redoing it and redoing it, and it's this perpetual cycle of build the platform, try to move their data to it, and then like five or six others pop up elsewhere. So it's a bit chaotic. A lot of it is focused on copying and pasting data around the organization. So they're probably spending more of their time doing plumbing than actually getting any real tangible value out of it.

Rajeev Nayar: It's a mixed bag, Chad. Basically we have enterprises that are pretty advanced in the way they're using data, and most of the enterprises are at a stage where they're trying to bring their data together to actually drive some of these AI initiators on the company. There is a focus on centralization. There are a lot of companies that are trying to centralize the data, which was a process for the longest time. Now it has reduced a little bit. People are okay to work with data that is federated, but being able to bring it together in a common way. What is missing over there is when we think about particularly driving AI initiatives, we need to do a lot more. We need to work with what we call multimodal data, which includes video, audio, and everything else. Most people have not really given that a lot of thought, how are they going to do it, how are they going to secure it, how are they going to manage it? And there's a lot of effort that is going on in that. So we are trying to help them with things like fingerprinting the data, getting this corpus ready. But a large number of customers are in that process of actually trying to get their data ready so they can trust it to be able to drive AI initiatives.

Chad Watt: What are some characteristics of that other 15%, the organizations that are making the most of their data?

Dael Williamson: So those organizations typically have brought data to the core of their business rather than having it in silos on the periphery. They're able to do quite fascinating things. So they're the fast movers in the sort of adoption of AI. And when I say AI, it's probably they've been using machine learning for many years and they're taking advantage of some of the newer waves like generative AI. They understand the future is more open, so there's an inherent interoperability. They are multi-cloud ready, so they can kind of move their data, they can read data from different sort of areas. Other characteristics is they've simplified their architecture and their technology environment massively. It doesn't mean that the tools that they're using are simple, it just means that they're not using 15 of the same tool in different domains. So those typically are the characteristics.
There's a huge inherent education of data to the people, because they use it and they create value out of it. And often what we see is they outperform their peers. In some cases, I mean we've tracked indexes of data that sort of cohort against their peers on like S&P and you can see I think one of the statistics we kind of calculated was a 30% outperformance. So it's significance in terms of how it can bring value. The reason we thought it was quite cool and what the observation that gave Databricks as founders their way of moving forward, was we saw all the Silicon Valley, Bay Area, Magnificent 7 companies, this is how they do things and we wanted to make that more accessible to everyone.

Chad Watt: And you're saying there's a direct correlation between high performance with data and outperformance in terms of business outcomes and enterprise value.

Dael Williamson: Otherwise, everybody's using intuition to make decisions. But when you're using both data and intuitions, that's a powerful combination.

Chad Watt: Rajeev, let me ask, is there anything you would add to that list of characteristics?

Rajeev Nayar: People are working a lot with data, but the real value, and this is something that we need to understand, people don't care as much about the data as they care about the insights through which they can take action, and that cycle is what we are trying to promote and we are trying to help customers with. We see a lot of people still stuck in the data engineering side where you are trying to bring this data together, but the real value is in getting the insights and taking action out of that.

Chad Watt: Let's double click down on data in Europe. We've had the GDPR privacy provisions, and in January this year, DORA, the Digital Operations Resilience Act, went into effect. How are companies faring in terms of data governance and compliance?

Dael Williamson: GDPR kind of came into in effect a good six, seven years ago now, and I couldn't tell you that governance is really concrete. It's still very fragmented. Data's still in silos. They have a many tools, many software product problem, which means that adhering to some of the newer, even the sort of GDPR legislation's hard, adhering to what's coming with DORA, that's going to be really difficult for a lot of companies. What it is going to do is motivate them to change. How they change, that's another thing entirely, and that kind of Rajeev could probably speak more to.

Chad Watt: So Rajeev, what's the biggest challenge here in these kind of compliance and privacy requirements that have been put in place?

Rajeev Nayar: GDPR people have worked through this and have a reasonable amount of control on it, because of the regulatory fines and other things that are associated with it. The ones with the AI regulation, one of the things that we have to commend is that Europe has been a leader in bringing these regulations to the forefront. So the problem is understanding those regulations and then applying it in a consistent way. If you think about it, you're trying to apply regulations on how you use AI. When AI governance is not defined, how do you govern it, how do you measure it so that you can have some amount of control on it. It is not defined.
So people are going to have to accelerate that process. They have to accelerate the process of how they're implementing AI in their organization, what is the kind of observability they're going to put in there, and how they're going to govern it. Because otherwise just implementing these regulations is not going to be easy. Compounders, you're talking about Europe, but compounders with 37 countries that are going to come up with regulations. In the case of US, every state is thinking about their own regulations. So this is a pretty complex problem. We think about big companies that we work with, which is international, now you can see the complexity of the problem. So I think the first steps is actually to get control on what we call their AI foundation and then put the AI governance in place, so that they can control what gets rolled out, and the veracity and the trust on the insights, and other things that are produced from that before they can actually support this entire regulation in this region.

Chad Watt: Dael, can you expand a little bit further on. What does it take for large organizations to gain value from their data?

Dael Williamson: So our belief and what we've proven, we have 12,000 customers and a lot of them on a journey, some have really fast moved to a far more sort of interesting promised land. They adopt something what we call the data lake house architecture. In effect it's a construct that has open standards and principles to systematically remove silos, and sort of get your data into a standardized state. And I'm not saying centralized, it's not sort of a centralized approach. It's more standard formats and it has a unified governance framework, which we call unity catalog, that allows you to get more out of your data. You still apply governance, it's just because it has standards, and famous quote by one of the VCs named Bill Gurley, he said, "The most complex problems in the world are solved by open source." And this is one of those incredibly complex problems.
What happens if you have open source tools at your base, you're able to apply governance, you're able to organize your data into a standard format, you're able to enhance discoverability of data, you're able to manage lineage of data, which is basically able to trace where it comes from, and you're able to apply access controls in an inherent way. And there's a lot of other things. You can apply the policies, that to Rajeev's point, like multi-jurisdictional policies that are quite different in some markets, those can be pushed down and applied in their sort of necessary way. So that's the kind of fix. It's a journey and it's not trivial, and there's a lot of baggage and decluttering that they have to do. But what we're seeing is massive success from those that are embracing that and sort of moving away from the sort of more legacy constructs.

Chad Watt: Rajeev, let me ask you first, have AI and generative AI helped companies do better with their data the way Dael's describing?

Rajeev Nayar: So I'd actually sort of reverse the question. The thing is, for AI and gen AI to do well, it requires a foundation of data that we say is AI ready. So the more you focus on bringing that foundation together, the more is the value that you can get out of AI engineer initiatives. Has AI engineer AI made a difference? Absolutely. I mean, look at coding for example and taking forces as an example. We use a lot of gen AI to do our bread and butter coding at this point. There are cases where we have got productivity of up to 80%. So these are significant changes. And in India, think about gen AI and how it has democratized access to AI across our customers and our organizations. It's amazing, but it has to be supported by a very strong foundation. You have to have trust in the answers that it provides. And there are variations, there are shades of these solutions. Gen AI doesn't equate to foundational models. I want to be very clear about that. So there are shades of these solutions and the main thing is the trust. And we had our own AI surveys that we do every year, and one of the main things that the executors have been telling us is trust in these solutions is one of the major factors in terms of adopting it.

Chad Watt: Very true. Dael, you want to add anything there?

Dael Williamson: Yeah. So I think my favorite quote that I've heard in the whole time of AI, other than the fact that makes natural language the new programming language, which is Andrej Karpathy, is that AI is a sophisticated mining equipment for haystacks. So what I mean by that is it is incredible at mining through large volumes of data to try and derive, and find, weak signals and insights from that. So to double click on Rajeev's point on code, and we were talking about this before we kicked off, it's incredible at reverse engineering very old code, and then it's incredible at converting that into new code through what Rajeev pointed out is like an 80% productivity gain.
So what it helps with, if you go back to how that process would've worked before, you'd have a whole lot of humans like mining through and reading line by line code. The same thing happens with documents, going through large volumes of documents. And I've heard some incredible use cases of very specific models that are trained for particular document banks. One trained on empirical evidence found the first antibiotic in 60 years. Another one has reduced the cost of looking for a particular type of concrete through building survey documents from six month process to a one week process. And the only reason that the one week is in existence, it wasn't like we couldn't get outputs faster, it was that we'd need to mark the homework. So there's material gains in how these tools work. It still means if we're going to use it sort of more on the output end, more on the distribution end, we need quality data going into it. So it is both a tool to help us mine and also a tool to help us accelerate how we engage with technology.

Chad Watt: In preparing for this, you mentioned something that I had kind of forgotten. Data has mass. That's a terrific reminder that somewhere behind all these virtual layers, there's something real electrical perhaps going on. Why is it important to remember that all these petabytes and exabytes have some real mass in kilograms?

Dael Williamson: Have you ever copied a large file from your computer to a storage device or cloud storage account? You always notice this takes time. It's like you've got the little thing going across the screen that's giving you the percentage of how long it takes to complete. The bigger the file, the longer it takes. Now take that thinking and apply it to how data flows in an organization in terms of that kind of exhaust and how it's moved around. That organization surrounding ecosystem also has the same inherent problem, how data flows between suppliers and that organization customers, and that organization partners. Every time a copy is made, we lose time. I'm a big believer that time is finite and time is one of the most valuable resources that you just can't get back. Nobody can make more time. I mean maybe somebody will figure out time travel one day, but let's assume that that hasn't been done.
Currently, we also lose more time due to the proprietary nature of data, and the checks and balances that have to be done to ensure the copy was successful and it's maintained what we call data integrity. So it says what it said when it was created. This is grossly inefficient. And as a biochemist, I look at the analogy of how a red blood cell gets copied just with precision. In this minute that I've taken to explain this, you have basically got about 2.4 million red blood cells have been copied in your body. And that's 3.6 billion nucleotides copied with absolute precision, and if that's not done accurately, these things develop mutations and you end up with pretty bad things happen to you.
Yet when you look at how we move data around organizations and how things work today, there's so many copies, so many checks and balances, and we just slow businesses down. And the older the business, the more steps in the chain, the slower the business runs. That's why we philosophically believe that the solution to this problem is open source, because it brings about open standards and it drives a higher interoperability, and it means you can actually have less copies because it facilitates a way to reduce those copies. And just to be clear, the approach is still governed, it's still secure. And what we've set about is building a sophisticated platform to optimize this approach.

Chad Watt: So what you're saying is open source is essential to what you're doing. How does that tie into the mission of democratizing data in AI for Databricks?

Dael Williamson: Basically, if you look at open source, open source when done well, and we've donated many projects to various open source foundations, when done well, you form a community and you form a healthy contribution base to those projects. It effectively becomes submissions in the greater mission. And the whole thing about democratizing as a mission and the analogy to open source is, open source effectively allows for democratic contributions. Traditionally in tech, if you wanted tech extended, you had to go join an advisory board and you would have to write down your requirements, and you would have to wait many months. Now, as an engineer who uses open source and open source based products, you can dive straight into the code, you can now do this at 80% more proficiency, and you can accelerate new contributions to that code and effectively extend it in the direction that you want it to go. And philosophically, that changes the game. Now, if you apply that to how you store data, how you access data, how you govern data, each of which is an open source tool in its own right and an open source project with an old community, you create a standard that can get adopted by everybody, and that is our mission.

Chad Watt: Is there some danger in democratizing data and AI? What are the guardrails to stay away from those?

Dael Williamson: So I think the danger is people are always going to do things for bad purposes, that started with the invention of fire. So I think there's always going to be unintended consequences. We work quite hard on actually creating guardrails and facilitating tools that help with guardrails. I think Rajeev probably could speak to this in a more detailed way and more in an applied way, like how we're sort of seeing join customers do this, because it's important.

Rajeev Nayar: Thanks, Dael. And the thing that we have to understand, I'm a believer in these open standards and open source side as well, but we have to understand what we're talking about. We have to understand that we are talking about the process, we are talking about the function, and not the content. Open sourcing data doesn't mean that the content is open to everybody. Open sourcing data for things like for example, creating open standards at the storage level so that we get away from these migrations that we are doing for the longest time, which adds no value to anybody, to be able to get away from that. Governing things in a consistent way, I don't see real value with everybody discovering new ways of governing something.
So we are talking about standardization of those capabilities, the content. And this is a very important thing to understand when you talk of getting enterprise data ready for AI. One of the things is... And I'll take a small example in the context of your large language models. Usually in an enterprise, you sort of govern a document. Based on the entire document, you put these digital rights management around it. When you talk in the context of a LLM, you're breaking up the document to what we call chunks. Now these chunks need to be governed, not just the entire document. So you have different sort of considerations that need to come into the picture, not just what we're used to. They get refined in an AI world and new sort of capabilities need to come in. So the content needs to be managed and governed at an enterprise level, and that is an important aspect. While the processing of the data, it should be based on open standards and open source so that we can actually benefit from the knowledge of the collective.

Dael Williamson: I'd just like to add one last point to that, because effectively that facilitates interoperability. And I'm going to take us all the way back to the exhaust example. If you think about driving a car, so I'm in the UK, you're in the United States, we drive on one side of the road, you drive on the other side of the road. If you flew to Heathrow and rented a car, your contextual rewiring would take two seconds. You get in the car, you start the engine and go. You just have to remember that you're driving on the other side and sitting on the other side. But the car itself is relatively interoperable in terms of how it works, what it does, how it functions, and all of those sort of things that you inherently know how to do as a licensed driver. That interoperability is what we're trying to achieve in the same way with open standards, and that's sort of why it's important.

Chad Watt: Dael, Rajeev, thank you for your time today.

Rajeev Nayar: Thank you, Chad. It was my pleasure.

Dael Williamson: Thanks, Chad. It's been amazing. Thank you.

Chad Watt: This podcast is part of our collaboration with MIT TechReview. In partnership with Infosys Cobalt. Visit our content hub at technologyreview.com to learn more about how businesses across the globe are moving from cloud chaos to cloud clarity. Be sure to follow Ahead in the Cloud wherever you get your podcasts, and you can find more details in our show notes and transcripts at infosys.com/IKI, that's in our podcast section. Thanks to our producers, Christine Calhoun and Yulia De Bari, and Dode Bigley is our audio engineer. I'm Chad Watt with the Infosys Knowledge Institute signing off. Until next time, keep learning and keep sharing.
About Dael Williamson
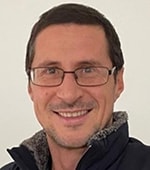
Dael Williamson is EMEA CTO, Field Advisory and Engineering at Databricks. Dael is an accomplished analytical technology leader, frugal innovator and chief architect with 24 years of commercial experience in technology, driving architectural vision, design thinking, as well as leadership on digital, data and AI transformations.
On LinkedIn
About Rajeev Nayar
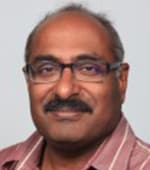
Rajeev has over 20 years of experience in the IT industry and serves as the CTO for the Data & Analytics practice at Infosys. His expertise lies in developing customer-centric intelligence solutions with a focus on AI, cognition, and smart, scalable data solutions.
His key areas of focus include AI, advanced analytics, data on the cloud, large-scale data solutions, big data, and emerging technologies for data processing.
He has been driving the strategy for developing cognitive solutions such as the Digital Brain. Additionally, he has built solutions for modernizing data landscapes and monetizing data assets for large global customers. These transformative solutions leverage the technology pillars of cloud, big data, and analytics. By harnessing these technologies, he has created a set of assets that differentiate the Data & Analytics unit in delivering value to its customers.
On LinkedIn
About Chad Watt
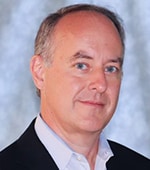
Chad Watt is a researcher and writer for Infosys Limited and its thought leadership unit, the Infosys Knowledge Institute. His work covers topics ranging from cloud computing and artificial intelligence to healthcare, life sciences, insurance, financial services, and oil &gas. He joined Infosys in 2019 after a 20-plus years as a journalist, mostly covering business and finance. He most recently served as Southwest Editor for a global mergers and acquisitions newswire. He has reported from Dallas for the past 18 years, covering big mergers, scooping bank failures and profiling business tycoons. Chad previously reported in Florida (ask him about “hanging chads”) North Carolina and Texas. He earned a bachelor’s degree at Southern Methodist University and a master’s degree from Columbia University.
On LinkedIn
- “About the Infosys Knowledge Institute”
- MIT Technology Review
- Databricks
Mentioned in the podcast