Foreword
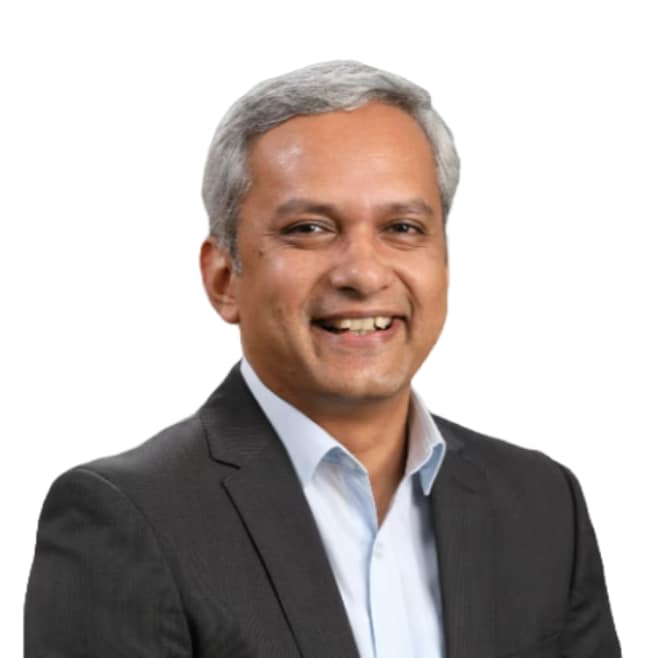
Rafee Tarafdar
Executive vice president and
chief technology officer, Infosys
The transformative potential of artificial intelligence (AI) has captivated everyone for decades, evolving from a futuristic concept to the pinnacle of the hype cycle. Now, AI is on the verge of its next level of evolution: Agentic AI. Unlike traditional AI, which enhances human tasks through insights and automation, agentic AI redefines both expectations and capabilities. It goes beyond supporting existing workflows, instead reimagining and redesigning processes from the ground up to create truly AI-native systems.
In its early stages, generative AI primarily served as a tool to enhance efficiency and accuracy for individuals and teams. However, as AI models have matured and become increasingly commoditized, the focus is shifting from augmentation to reinvention — transcending incremental improvements. This transformation echoes past technological revolutions, such as the shift to digital-first processes during the rise of digital transformation. Just as businesses once reengineered processes and business models, rather than merely digitizing analog workflows, agentic AI demands a similar rethinking of processes to unlock its full potential. Achieving this requires deep integration of cognitive capabilities into process engineering and experience design — not just at the periphery of business but at its very core.
The potential of agentic AI extends far beyond its early applications in customer service and IT operations. It has the power to revolutionize mission-critical domains, such as customer onboarding and credit decisioning in banking; supply chain management in retail, consumer goods, logistics, and manufacturing; sales and marketing lifecycles; and product design and development. By embedding intelligent agents across these functions, organizations can unlock higher levels of efficiency, adaptability, and innovation, fundamentally transforming how they operate and compete in an AI-driven world.
Yet this journey is not without its challenges — the inevitable barriers, frustrations, and setbacks that mark all progress. The technology is still evolving, and enterprises face critical questions about how to strategically implement agentic AI at scale. How should organizations architect systems that can accommodate hundreds — or even thousands — of intelligent agents? Which platforms and models should they adopt? How can they ensure interoperability among diverse AI systems while maintaining flexibility for future advancements?
This report explores these critical questions through a pragmatic lens, cutting through the hype surrounding agentic AI to provide actionable insights for enterprise leaders. It offers practical guidance on navigating this complex landscape and implementing AI-driven strategies effectively. By adopting a poly-AI and poly-agent architecture — one that integrates the best models, providers and agents while still ensuring interoperability — organizations can stay agile, future-proof their investments, and gain an early lead on competitors.
Chapters
Agentic AI is transforming artificial intelligence by enabling autonomous decision-making and real-time adaptation with minimal human input.
Unlike traditional automation, it can reason and act independently, making it a valuable business partner. Large language models serve as its cognitive core, unlocking the advances to come.
Agentic AI has been called the top technology trend for 2025 and is predicted to continue its strong growth in the near term. About half of enterprises that use generative AI are expected to adopt agentic AI by 2027. As businesses explore this technology, agentic AI is expected to reshape the way enterprises approach their operations, much like generative AI has upended industries and functions.
Agentic AI is transforming decision-making and workflow automation by enabling autonomous agents to perceive, plan, and act within complex environments. Its effectiveness depends on understanding agent roles, capabilities, and the seamless integration into business processes.
The sense-plan-act cycle is central to its architecture, ensuring transparency, adaptability, and collaboration with human teams. Choosing the right AI agents — from simple reactive systems to advanced learning models — is crucial for optimizing efficiency and innovation.
A well-structured agentic AI system enhances scalability, flexibility, and continuous learning, providing businesses with a sustainable competitive advantage while maintaining reliable and intelligent decision-making processes.
AgentOps is a lifecycle management framework that streamlines the design, deployment, monitoring, and optimization of agentic AI systems. Building on DevSecOps, MLOps, and LLMOps, it addresses the unique challenges of AI agents, ensuring scalability, reliability, transparency, and efficiency.
This framework incorporates governance measures and guardrails to maintain control, enhance behavioral consistency, and prevent unintended actions. Despite its benefits, AgentOps remains underutilized in generative AI, potentially limiting AI’s impact.
By adopting AgentOps, organizations can reduce failures, accelerate issue resolution, and optimize AI performance, ultimately unlocking AI’s full potential in automating enterprise operations while maintaining trust and accountability.
Organizations that adopt AI agents need a strategic approach that extends beyond technology to effective development and execution. These agents support IT operations, customer service, and content management using diverse reasoning models but face challenges like memory management and efficient information retrieval.
Overcoming these issues is crucial for AI agents to provide accurate, context-aware responses and automate processes effectively. Frameworks like MetaGPT, AutoGen, and CrewAI enable agents to collaborate and operate in parallel, improving their efficiency. Breaking down user requests into clear, step-by-step plans ensures AI agents deliver reliable, timely, and contextually relevant outputs across enterprise applications.
Organizations are integrating responsibility into agentic AI systems used in complex fields like healthcare and financial trading. However, 72% of AI practitioners identify the lack of rigorous evaluation methodologies as a major barrier to trustworthy deployment.
Ensuring responsibility in AI requires embedding ethical frameworks into its architecture, supported by innovations such as neuromorphic ethical models and federated fairness audits. Yet, these systems face challenges, including dynamic value drift, adversarial robustness gaps, and reasoning limitations in ambiguous scenarios.
Effective AI governance demands aligning autonomous systems with evolving human values through technical innovation and collaborative oversight, ensuring reliability, fairness, and ethical decision-making.