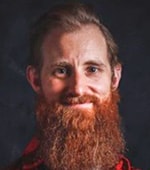
Dr. James Fergusson, Associate Professor, Centre for Theoretical Cosmology, Executive Director of the Data Intensive Science group including the Centre for Doctoral Training and MPhil in Data Intensive Science, and Director of the Infosys-Cambridge AI Centre shares the research work done in the centre during a recent interaction. Multi-agent systems, AI-enhanced simulations, and mathematical AI are the themes behind the AI Centre. He shares his thoughts on businesses realizing an AlphaFold moment with AI.
The AI Centre in the University of Cambridge was developed after seeing the transformation coming in science, driven by the exponential growth in data, compute and AI, and the need to upscale PhD students and postdoctoral researchers with these new skills that they do not get in a traditional degree. There is a transformational opportunity to change the way research work in science is done using AI, says Dr. James.
Themes in the AI Centre: There are three main themes that are looked at in the centre: Multi-agent systems are a way of automating a lot of the work that a PhD student would normally do: processing the data, building the software, writing things up, generating plots. The centre has built large multi-agent systems where questions about the data can be asked, and it will go all the way through the analysis of the data to writing drafts of the paper with the results. The speed of innovation could be rapidly accelerated in many different sectors.
The second theme the centre is interested in is AI-enhanced simulations. Scientific research is dependent on simulations, both to better understand physical systems and to quantify errors in our measurements. A very large number of simulations are required for this purpose, to understand not only what is measured, but how sure of the outputs we can be. And that is very computationally expensive.
Cambridge has been a very early adopter of high-performance computing for this reason, with AI seen as a way of accelerating a lot of this work. There is the Polymathic AI project, an international collaboration that is working on building a foundation model for numerical data. This is like a ChatGPT but for numbers. A huge data set of all different types of data is built — images, time series, simulations.
The third theme is mathematical AI, or theoretical physics for AI which looks at two main topics:
- How do we take ideas from theoretical physics and use them to understand how neural networks work and how they learn. An analogy for this is when we had the industrial revolution, we built engines, but we couldn't manufacture efficient engines until we developed thermodynamics.
- How do we extract knowledge from ML systems. When we train machine learning on data, it is learning something, but it doesn't tell us what it is. Somewhere in that black box is hidden the knowledge of how the system works and key focus of our research is on how we can extract that knowledge back into a human understandable form, in either equations or code. One example where we have demonstrated this is with all planetary orbits data which is fed into a neural network which is trained to predict the next time step. We can then use methods like symbolic regression to extract from the neural network the equations that govern the system, in this case being Newton’s inverse square law for gravity. This allows us to progress from data to knowledge in an automated way. This works with any system. For example if we have a large black box AI system and it performs well, we can say, can I approximate that with equations? The equations will tell us not only which features are important, but also how they interact with each other to give the answer.
An analogy for mathematical AI or theoretical physics for AI is thermodynamics. When we made internal combustion engines, we could not make them efficient until we developed thermodynamics.
Collaboration with Infosys: Every industry, sector, and business has seen exponential growth in data in a data rich world. How do we harness that for knowledge? This is why the collaboration with Infosys was created in the AI Centre, because the problems we are trying to solve are the same. Many of Infosys’ clients want to have explainability. They want to have simulations that can run faster and can be trusted. They want to use the power of multi-agent systems to automate business processes for knowledge processing tasks.
As part of this collaboration, pure scientific research that has very wide applicability will be carried out. Infosys is the connector between the centre and the business world, so that this knowledge can be shared globally across all sectors.
Humans in the loop: The question of humans in the loop in AI implementations has a lot of hype and discussion. Every time we build a new IT system that does something, it requires supervision and maintenance. We have never built a technology that can work perfectly in a vacuum by itself, says Dr. James. If technology can automate key tasks in a sector, it is an exaggeration that everyone in that sector will then lose their job. While ChatGPT is fun to play with at home, would we want to use it unsupervised it in a business-critical situation? We can't take the risk of leaving our business in the hands of a technology that doesn't really have any boundaries or checks on it, because mistakes are very, very costly.
Every time we build a new IT system, it requires supervision and maintenance. We have never built a technology that can work perfectly in a vacuum by itself.
There will always be the need for domain expertise, for people to validate and check the models we build, to maintain and update them, and also to observe the decisions they make. The skill sets will change, and maybe the number of people in each area will change. But it is a hyperbole to anticipate this world where there is going to be a company that has only six people with everyone else replaced by AI.
Business value from AI – an AlphaFold moment: Businesses have not yet realized value from their AI investments, says Dr. James. AI is definitely exciting, and it's fun to play with. But we haven't got past that barrier where AI becomes a serious tool for decisions. AI should not be just for fun. It should do critical things that we can trust, believe in, and understand. We are very close, and we will see in the next one to two years some real key cases coming through, announcing that we have done this in a serious way that really changes the way we work.
We have one example in science with AlphaFold, an AI application to predict protein structure. That is an application that has been done very seriously, very reliably, and has broken a problem that was otherwise intractable. This was the first case of AI driving real scientific knowledge and changing the way a whole field works. The broader science community now can see the proven value, and AI is being integrated in many research areas. In businesses, there will be the AlphaFold moment where a business comes along and says, we have cracked automating or accelerating some large part of a business with real tangible bottom line benefits. When that happens, that's when the floodgates will open because someone will have proved in a concrete way how to harness AI to deliver real value that is not limited to the very specific narrow problems where it is being used now.
Organizations that are primarily into information processing are the ones where there is real benefit to AI. If parts of such a business can be successfully replaced with AI with trust and in robust ways, that has a real transformative effect.
How can abstract theoretical physics be useful with concrete technology? The Nobel Prize for Physics in 2024 went to AI, for the statistical mechanics that underpinned some of the design of it. It is always fundamental. At the moment, AI is a lot of trial and error. What is currently missing is the foundational theory of when you build a large network, these are the key things that help it learn well. But we don't really understand why.
And theoretical physics has a real long history of dealing with this kind of system. It's very similar to a system of particles in a gas chamber for example. We are looking for collective variables, or collective descriptions of complex systems, which are actually individual neurons interacting with their local neighborhood.
Most AI systems mimic human behaviour – a system that detects or labels images like a human for example. But how do we actually turn it into generating knowledge or understanding, so that when you load customer data, it predicts whether the customer will leave the bank. Predicting something is great. But what do we do with that if we don't understand why it is happening? How do we make a business decision about how to address the fact that a customer is leaving? We need an understandable model that says a customer is leaving because of this particular reason, which you can then act on, says Dr. James on a closing note.