Insights
- Many businesses have not prepared the ground for deploying AI.
- Third-party data, gathered from a wide range of sources, offers promising insights but is also a challenge.
- There is a tension between using data for new purposes, and the ethical use of that data.
- Data maturity helps companies deliverer superior customer experiences.
If 2023 was the year of experimentation with artificial intelligence (AI), 2024 was about moving to the next stage — finding and delivering value from AI — and 2025 will be about building on that. However, before that can happen, businesses need to make sure they are AI-ready — and many enterprises are not yet there.
Research by the Infosys Knowledge Institute identified five pillars of readiness that enterprises must achieve in order to deliver on their AI ambitions: strategy, governance, talent, data, and innovation. And yet of the 1,500 companies around the world who were surveyed for the research, only 2% had all five of those foundational building blocks in place.
On data, no more than 13% of respondents were confident about the accuracy of their corporate data, their ability to locate data, governance, and their ease of access to it, with up to 37% of respondents worried about accuracy, governance, ease of access, and their ability to locate their data (Figure 1).
Figure 1. Challenges to AI adoption
Source: Infosys Knowledge Institute
Also at the heart of AI readiness is ethical AI, and again, data is a foundation stone. Infosys distinguished technologist Rajeshwari Ganesan told the AI Interrogator podcast that AI systems must take an ethical approach from the very start: “We need to begin early, and we need to engineer this in the lifecycle state. Responsible AI has multiple dimensions: it may be safety, it may be reliability, it may be fairness, ethics — we can composite it in one phrase called responsible AI.”
Ganesan pointed to the need for transparency about how AI generates its outputs, or what’s known as explainable AI. Again, this depends on being clear not only about the algorithms that are used, but also about the data that underpins those outputs. She explained: “The most interesting case, which I’ve been fascinated with, was when lots of data was fed into the systems, they were able to find the gene expression related to triple-negative breast cancer. Which physicians did not know to begin with.
“But it was a double-edged sword. On one hand it was finding patterns, but how do you know if these patterns are right or wrong? This led to a situation where these models were ‘black box’. So whether it came to loan delinquencies or whose resumes were picked up by the system … there was always this concern that these black-box models; we had to have better explainability.”
Enterprise AI depends on a data architecture that can handle a huge range of data types, both structured and unstructured. Data that feeds into AI is not only text, audio, and video, but can also be user-generated data, synthetic data, telemetry data, and third-party data.
Third-party data can be particularly challenging, as the enterprise might not have insight into how it was gathered, and into safeguards for governance, compliance, bias, security, and privacy.
Third-party data can offer deep insights — something marketers in particular are benefiting from, as the Infosys CMO Radar 2024 has found. The report, which spoke to 2,600 marketing leaders across 14 industries around the world, found that 73% of marketers have experimented with or already deploying AI in their marketing activities, and that AI is used in nearly 60% of marketing personalization, campaigns, and content creation.
This makes it even more essential that the data underpinning this work is suitable and has sufficient guardrails around it.
Speaking to the AI Interrogator podcast, author and journalist Timandra Harkness pointed to the New York City Taxi & Limousine Commission’s public dataset, which is freely available. “You could go into that dataset now and pick out an individual taxi in the past, and see where they picked up, and where they went, and where they dropped off, and what fare they got.
“Perhaps more worryingly, if you could follow a taxi driver’s regular habits, then you could work out perhaps this taxi driver regularly stops five times a day at the Muslim prayer times. So, you could actually deduce very personal information from people from a dataset that had been made public because taxi meters are a public information source.
“So, there is always a tension between using information for a new purpose that might let you do wonderful things, but if somebody hasn’t consented for their data to be reused for those purposes, it could have consequences for them.”
Infosys research has found that data readiness fuels the best results from AI: The CMO Radar found that enterprises with advanced data architecture and maturity in data are the ones that deliver the kind of superior customer experiences that drive growth.
The research identified three groups among marketers: Laggards, who have a reduced chance of achieving value from AI because they are rushing ahead without sufficient planning; Learners, who have had some AI success but could improve their AI fluency; and Leaders, who have established the robust frameworks and processes necessary to deliver on the promise of AI.
A priority for 2025, as enterprises move beyond the hype and focus on delivering value from AI, must be to make sure that their data is ready to serve as a foundation stone for their success.
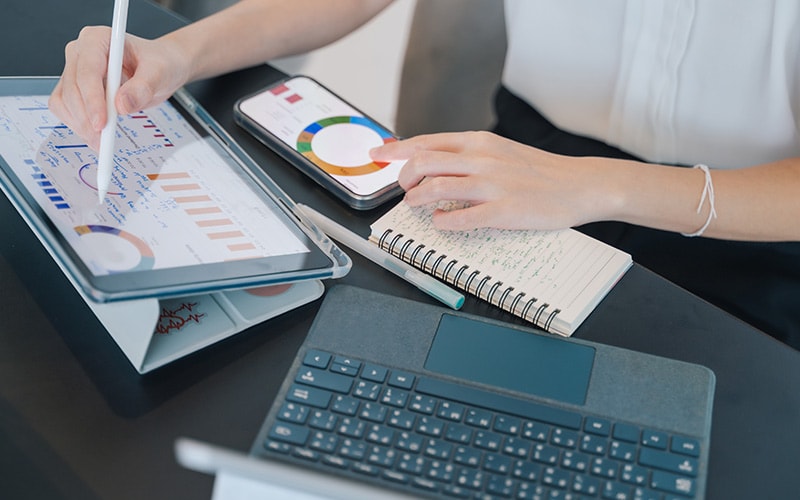