Insights
- Synthetic data is used in everything from generative AI, robotics, the metaverse, and 5G.
- Risk of bias, inaccuracy and privacy apply to synthetic data.
- Research shows synthetic data will overshadow real data by 2030.
- Any use of a dataset, real or synthetic, will fail it if does not account for the human factor. The principles of value, privacy, ethics, and sustainability must be considered while generating synthetic data.
- To use synthetic data responsibly and effectively, enterprises should build synthetic data centers of excellence.
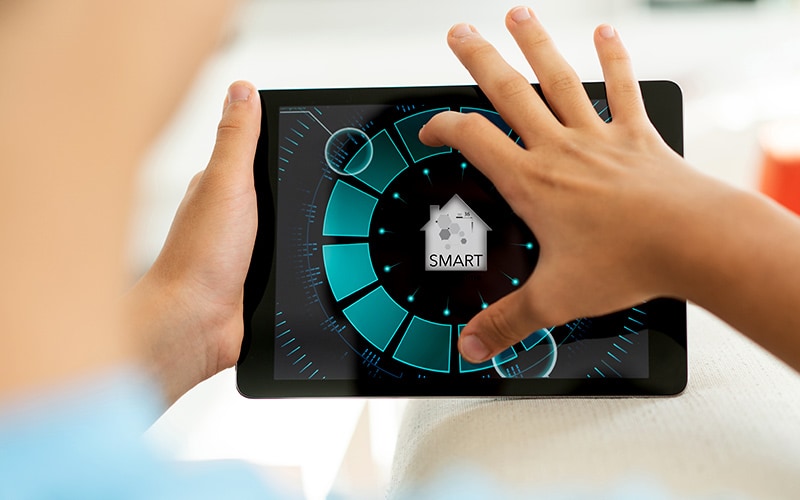
Enterprises have been generating and using huge volumes of data for more than two decades: in 2020 alone, we generated, copied, and consumed 59 zettabytes of data – enough data to fill about a trillion 64GB hard disks. If these hard disks were stacked as a brick wall, it would be longer than the Great Wall of China.
Today data passing between countries is regulated as if it were a physical commodity, even though data needs no passport or transportation beyond the internet superhighway. Enterprises and countries alike realize the real value of data in the form of ideas, talent, and inputs that spur innovation and productivity. Hidden within these vast volumes of data are insights into consumer behavior, emerging market trends, even predictors of the future.
The dawn of enterprise AI has shifted the focus even more towards data for decision-making. However, enterprises do not always have suitable data to train and improve models, and so many turn to synthetic data to fill the gaps.
However, synthetic data brings its own problems, including risks of privacy, bias, and inaccuracy. As Rajeev Nayar, CTO of Data & AI at Infosys, states: “In the AI world, you will not achieve enterprise scale without synthetic data, but the question is how you manage it. And how do you mitigate bias and the larger second-order effects.”
Enterprises therefore need a set of principles and a framework to mitigate the risks by prioritizing humans and their data over business needs.
What is synthetic data and why a growing interest in it?
Synthetic data is computer-generated data used as a replacement of data from humans or real-world events. Algorithms create data sets for testing or training purposes. Synthetic data can mimic operational or production data which is used to train AI in areas where real data is scarce, too sensitive to use, biased or has a risk of a personal data breach like medical records or personal financial data.
Synthetic data is a simple concept which is poised to upend entire value chains, business models and tech stacks for data-centric services with significant economic implications. Synthetic data is not a new idea but is approaching an inflection point of large-scale enterprise adoption with real world impact. Enterprises need a robust strategy to harness the power of synthetic data in their data arsenal.
There is a growing interest in synthetic data due to the factors in Figure 1, which we explore in turn.
Figure 1. Factors driving interest in synthetic data
Source: Infosys
Data hungry AI and the need for high value data
Modern ecosystems depend on data, and growing technologies like Generative AI, machine learning (ML), and internet of things (IoT) require massive volumes of data. This in turn demands data that’s accurate, up-to-date, and well-organized. However, this kind of data is difficult to acquire and to use – and it is also expensive. Research by the Infosys Knowledge Institute found that nearly 40% of enterprises struggle with the high cost of finding the right data for their AI initiatives (Figure 2).
Figure 2. 4 in 10 enterprises lack accurate data for AI initiatives.
Source: Data + AI Radar 2022, IKI
In 2001, Google engineers added a line of code that kick started today’s data-driven economy: Google Tag Manager. This code captures users’ personal information from searches, and then performs computational analysis to predict users’ preferences to deliver personalized ads and recommendations.
Enterprises have used this same approach to track users and generate data. It has revolutionized business, from customer service and employee experience to operations.
This abundance of data, in turn, has spurred AI experimentation in enterprises of all sizes. Indeed, analyst firm Forrester predicts that enterprises will spend around $64 billion USD by 2025 on AI tools, AI-centric software and AI-infused software. Consumers have seen the effects of this spending: algorithms are used for automation, facial recognition, driving cars, interpreting spoken language, reading text, drafting reports, grading student papers, and even setting people up on dates.
In theory, therefore, enterprises should have all the data they need for algorithms. However, two thirds of all data within an enterprise goes unused for decision-making, either because it is not accessible, or because it is poor-quality. Poor quality data includes not using a standardized naming process, with inconsistent formats for such things as times, dates, and addresses. Insights into consumer behavior, emerging market trends, and predictors of the future remain hidden within these vast, unusable volumes of data. In addition, poor data diminishes AI outcomes. Only 10% of enterprises report significant return on investment (ROI) from implementing AI (Figure 3).
Figure 3. Exponential data growth, yet poor ROI
Source: BCG
Case for synthetic data
Though the concept of synthetic data came into existence in 1930, it gained commercial adoption only in 2010, with the advancement of synthetic data simulation for autonomous vehicles and drones. The growing importance of computer vision, robotics, security, metaverse and smarter 5G networks pushed enterprises and academia to invest in enterprise-grade capabilities for synthetic data. MIT, which claims synthetic data as one of the top ten technology breakthroughs in 2021, has invested in Synthetic Data Vault, a project launched in 2021 by MIT’s Data to AI Lab to improve the adoption of synthetic data through open-source tools for creating a wide range of data types.
Synthetic data is increasingly widely used, in applications from robotics and security to smart 5G networks and academia. Indeed, Gartner predicts that synthetic data will completely overshadow real data in AI models by 2030 (Figure 4).
Figure 4. Synthetic data will overtake real data in AI models
Source: Gartner
Amplifying human potential
Synthetic data helps enterprises fill in gaps in their data and innovate – which means it has significant economic implications. What if we were able generate infinite amounts of the world's most valuable resource cheaply, at will? What economic, social, and business opportunities or impact would it have?
Today, synthetic data makes this a reality. It gives enterprises ability to create and build new business models based on artificially created data. For instance, humankind has been trying to build autonomous driving cars since 1930. One hundred years later, we are still way off from having fully autonomous driving vehicles which can navigate through traffic like a human driver.
Take, for instance, the data requirements for self-driving cars: a human driver not only has to know how to operate the vehicle, but must also be constantly aware of road conditions, other drivers, pedestrians, cyclists, the impact of the weather, as well as local traffic laws.
As Alexandra Ebert, chief trust officer, Mostly.AI, and chairperson of IEEE explains: “Synthetic data helps in training autonomous vehicles. Rabbits running on the road might not currently be an edge use case, but synthetic data could be a helpful approach to create millions of hours of diverse footage. A synthetic data generator using knowledge of physics can create realistic synthetic data and avoid expensive production of millions hours of video footage.”
Figure 5. Human reality vs. autonomous expectation
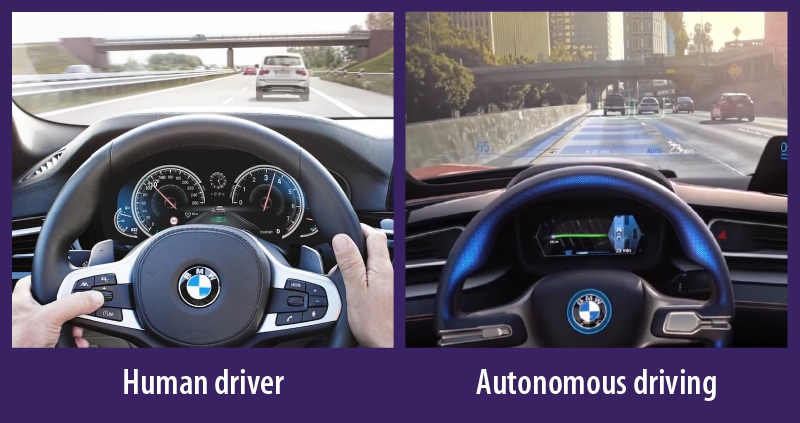
Building these intelligent systems requires enormous amounts of data so that the car can manage scenarios from congested cities to rural areas, from bright sun to driving rain. However, it is extremely expensive and difficult to collect sufficient data. Synthetic data can be used instead to model real-world driving situations, complete with people, traffic lights, empty parking spaces, and more. This is a good use case for synthetic data because it doesn’t involve mimicking data where privacy is a concern. Enterprises still need a rigorous approach to synthetic data in this use case, however – for instance, they need to make sure the data is close enough to real data to be useful.
Only a handful of enterprises can afford to produce and test self-driving vehicles, but those that can stand to gain significantly. By 2035, autonomous driving could create $300 billion to $400 billion in revenue.
Risk of synthetic data
However, with great possibility, comes great risk.
As Sanat Rao, CEO at Infosys EdgeVerve, states: “The growing concern is that the technology is moving very fast and the associated ethics framework and thinking around privacy, transparency, bias, and other softer aspects are not getting the right kind of attention.”
It is no longer a question of whether enterprises should use synthetic data or not – most already are. And the more AI they adopt, the more they will use synthetic data. This is a hockey stick moment for synthetic data, with a rapid uptick in the next few years. However, many are using synthetic data without a careful strategy to mitigate its risks.
The following risks are important to keep in mind:
- Value: Cost-incurred and the extent to which synthetic data meets business objectives are critical parameters to measure. Maximizing data value is one of the key objectives of using synthetic data. At the same time, enterprises should be aware of the costs and use the right techniques for data generation and scaling of synthetic data operations.
- Privacy and security: Synthetic data is an approximate duplicate of real data from individuals. However, because it is linked to real data, it is vulnerable to attack.
- Ethics: Synthetic datasets can be designed for fairness and to reduce bias. However, because synthetic data depends on original data, it can reflect the bias of the original datasets.
- Sustainability: Hoarding data and creating a lot of unusable data, whether real or synthetic, potentially increases energy use and carbon emissions.
However, these risks can be managed. Key to this is keeping the human dimension of data at the forefront of an enterprise’s approach.
Human-centricity of data
Data is often not used with people’s interests and needs in mind. Data exists independently of people, but it’s only valuable when there is clear understanding of how people will approach and use it. Products fail when designers do not consider the product’s human context, and the same logic applies to the design of datasets.
Any use of a dataset will fail if it does not account for human expectations. People now expect their data to be private and protected; data that does not incorporate protection and privacy creates reputational, financial, and legal risk.
Components of human-centric data
We believe that keeping the human dimension of data at the forefront is the best approach to mitigating risks. Figure 6 compares the traditional approach to data with the with the human-centric approach we advocate.
Figure 6. Traditional vs. human-centric data
Source: Infosys
Principles for using synthetic data
Taking to heart the above, we believe that enterprises should start with the following basic principles as they decide how to use synthetic data.
These principles are rooted in the understanding that data derives from humans.
Figure 7. Data with human-centricity, privacy, ethics and sustainability at the fore
Source: Infosys
- Principle 1: Prioritize people
Enterprises should put people at the heart of their data. They should know whose real data has gone into generating the synthetic data, and they should be clear that those humans have explicitly consented to their data being used in this way. Additionally, the enterprise should explain clearly how business needs take into account real humans. - Principle 2: Maximize the value of data
Enterprises should understand the cost and techniques needed to generate and scale synthetic data operations. For instance, synthetic data for a clinical trial could be reused, whereas real data created to test claims applications for the same set of patients has less data utility and reuse potential. - Principle 3: Safeguard privacy
Enterprises must comply with geographic-specific regulations for personal data protection and security, and each firm has different thresholds for acceptable privacy-protecting risk levels. Synthetic data can help here and has the potential to address complex privacy and security challenges. Synthetic data makes compliance decisions easier because it doesn’t contain information linked to real people. - Principle 4: Use data with responsibility
The principles of secure- and ethical-by-design apply to synthetic data: enterprises should be able to identify and correct data with ethical or fairness issues. Enterprises can more effectively validate synthetic data than real data for ethics and fairness. - Principle 5: Offset energy consumption
Generating and consuming real data is environmentally costly. Synthetic data offsets the environmental impact of ingesting, processing, and storing data. For example, a training model can emit more than 626,000 pounds (about 284 tons) of carbon dioxide equivalent — nearly five times the lifetime emissions of the average American car, including the manufacture of the car itself. Specifically, enterprises can build a do-it-yourself kit to create synthetic data and offset energy consumption. This will be a primary method to reduce the environmental impact of data-centric products and services.
Journey to synthetic data
Enterprises need to be acutely aware of the risks and problems of synthetic data. To work with synthetic data responsibly and to best effect, they should build synthetic data centers of excellence (COEs). These COEs support enterprises as they mature technology, processes, and skills to build synthetic datasets.
Enterprises can evolve their synthetic data capabilities through the three-phase maturity model described in Figure 8.
Figure 8. Maturity model of synthetic data program
Source: Infosys
- Stage 1 – beginner
At this stage, the enterprise has started working with experts to identify, examine, and quantify the advantages and limitations of synthetic data. The team frames the problems to be solved by synthetic data. The team identifies tools to generate synthetic data for use cases and the appropriate format. - Stage 2 – professional
At this intermediate stage of maturity, data scientists ensure the statistical validity of the sample and distribution of the synthetic data within the organization. The enterprise measures synthetic data ROI and refines the process of building synthetic data based on feedback from business functions and expert groups. They either deploy enterprise-class synthetic data platforms in the enterprise network or consume synthetic data generation services. - Stage 3 – expert
At this mature stage, the enterprise has a center of excellence that houses experts, who in turn train team members to monitor the generation and use of synthetic data. The enterprise coordinates with data privacy, security, and legal teams to understand the potential for synthetic data usage and create guidelines for its use. At this stage, the enterprise also has the technical expertise to build data pipelines for generation across multiple formats to create large volumes through automation.
Moving ahead
The total addressable market of data and the total addressable market of synthetic data will converge very soon. Enterprise must now think how best to embed this data in key operations, processes, and customer experiences. Whole industries will be born based on sophisticated simulation of real-world information.
As systems move from AI augmenting humans to humans augmenting AI and then onto AI twins and AI-powered ecosystems, the strength of a firm will be based on how much it is able to automate while keeping the human-factor clearly in mind.
Data, the driving force behind sentience and intelligence, has always been a mystery. Further, converting data into value in a privacy-first, ethical, and sustainable manner has always been a challenge for enterprises. Human-centricity in machine generated data seems to be a paradox, but data driven decisions on synthetic data without the right guard-rails will do more harm than good.