Insights
- The widespread adoption of AI brings forth ethical and legal concerns.
- Responsible AI should become a part of the entire development process and not just an add-on towards the end.
- More than legal enforcement, implementing stringent self-regulation will ensure ethical and unbiased practices.
- Larger companies sharing their AI best practices with the rest of the industry will ensure global adoption of ethical practices.
Artificial Intelligence (AI) has become integral to diverse sectors, from recruitment and healthcare to retail, banking, and financial institutions. The emergence of generative AI platforms such as ChatGPT has brought AI into everyday life. Across the board, the focus is to increase productivity with the help of AI. Yet, this widespread adoption brings forth ethical and legal concerns. The ubiquity of AI has necessitated not just a thoughtful but also a proactive approach from organizations for the responsible and ethical use of AI.
Responsible AI has emerged as an approach to address these concerns. It emphasizes that organizations involved in AI development and utilization must ensure that outcomes are unbiased, privacy-protected, and ethically sound. However, implementing this approach is not without its challenges.
Three industry experts – Vani Peddineni, group manager of Toyota financial services; Van Lindberg, a partner at Taylor English Duma LLP; and Miku Jha, director of AI/ML and generative AI at Google Cloud – joined Inderpreet Sawhney, Infosys’s group general counsel and chief compliance officer to discuss the challenges at an Infosys Topaz event on AI in Richardson, Texas.
Governance framework
While the concern that AI governance might hinder progress often arises in debates, there is now a consensus that appropriate governance is essential for ensuring the reliability, safety, and trustworthiness of AI tools.
Inderpreet Sawhney highlighted three types of possible regulations. The first is legal regulations imposed by traditional legal systems, which raise critical questions such as ownership of data, protecting AI-generated models, and the appropriateness of using data to train AI models. The second type of regulation is through self-governance, where organizations voluntarily adhere to ethical guidelines and best practices. The third is to regulate AI through contracts to enhance transparency and accountability. Sawhney then turned to Van Lindberg for his opinion on the most effective approach to regulating AI.
Lindberg said, “I think that there is going to be a mixed role for regulation. Legal, industry self -regulation, contracts - all three are going to interact just like they have. We had a lot of the same discussion around coming out of the internet. People were afraid of how it would work, different types of abuse, and we've mostly been able to figure it out with very little internet-specific regulation. I think it's probably going to be similar.”
Handling the AI regulations and accountability
With the EU imposing restrictions on companies adopting AI, the central question is: Who bears responsibility when AI goes awry? Additionally, how do companies navigate the constantly changing regulatory norms?
Peddineni shed light on Toyota’s approach: Toyota encourages employees to bring forth use cases spanning the entire customer journey. However, these cases undergo evaluation by a panel to determine their suitability for AI deployment as part of Toyota’s rigorous governance process to ensure data transparency. This includes meticulous filtering of the data fed into the model, ensuring accountability for the outcomes. She added “We put in extremely rigorous controls around data acquisition, protection, and utilization for various use cases.” Furthermore, Toyota prioritizes continuous education for individuals involved in AI and generative AI.
Legally binding assurances and self-governance
Large companies play a pivotal role in ensuring the reliability and safety of AI systems, employing stringent measures to navigate the complex landscape of AI solutions across varied domains. The task is challenging, especially when biases can be introduced during the pre-training of models. This underscores the critical responsibility of companies creating foundational models.
Sawhney’s next question was for Miku Jha. “As companies are putting out AI models, we hear a lot about responsible AI. These are business level assurances that companies are giving to their users, to their stakeholders. How are companies like Google thinking about it? And are they willing to sign on the dotted line and say, yes, we will stand behind these assurances and these are going to go beyond business assurances. We'll make sure that if we say we are responsible and our models are responsible, they're going to be legally binding warranties and indemnities that come with adoption of our models.”
Jha emphasized that organizations must establish robust AI principles for self-governance. “For us to get to any kind of a tangible solution for responsible AI, it has to be a multi -pronged approach. What are the AI principles for any given organization? Are you actually translating that from the word into some kind of a workable framework? That's hard to do because that means every single feature that you touch, every single data that you put into the model, every single evaluation that you make the models go through will have to cross check against those AI principles. And then you have to analyze the output - is the model safe? Is it responsible? Is it going to have a positive or a negative impact on the environment? Is it sustainable?”
Lindberg added that while safety concerns with open models are valid, it is crucial to consider the context when evaluating models.
Dismissing a model as unsafe without accounting for context can be wrong, especially since very few contextual assumptions are built in open models.
Sawhney highlighted the need for maturity in organizations regarding self-governance, questioning whether they are capable of strict enforcement, adding that self-regulation should not be confined to individual organizations but should extend to the entire industry. Peddineni concurred, saying: “We (Toyota) sell cars, we sell insurance products, we are now selling boats, mobility is our thing. So across industries, if companies like Toyota and other large companies come forward and start applying the standards globally, that's the way to go.”
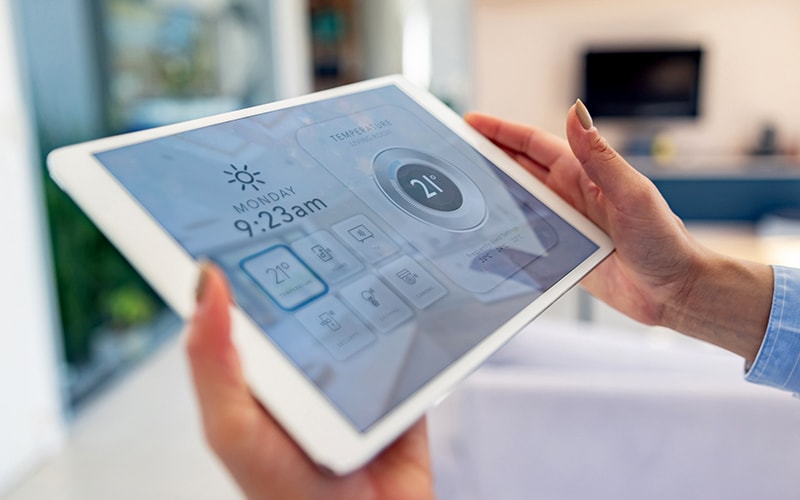
Responsible by design
Ethical AI centers around the concept of ‘responsible by design,’ which involves human oversight throughout the AI development lifecycle. It also mandates continuous auditing of processes to ensure fairness and transparency at every stage of AI deployment. Peddineni noted that companies must aim to establish best practices for ethical AI that can be universally applied and shared with others in the industry.
The debate over AI regulation is ongoing. While some argue that regulations hinder progress, the consensus is that a lack of regulation could lead to disastrous outcomes, such as biased decision-making or privacy breaches. Perhaps the most prudent course is to follow self-governance and adhere to responsible AI practices throughout the lifecycle of AI solution development.