Insights
- Tiny AI is one of applied AI’s most advanced trends yet. Complex algorithms run on edge devices themselves, and offer real-time predictive ability, all without the need for internet connectivity.
- In this paradigm, intelligence is aware, data is private, systems are secure, and personal assistance is instant, opening doors for new enterprise AI applications.
- However, not all current AI will work on the edge, and many applications and models still require the computational resources and processing technology provided by massive cloud data centers.
- These deficiencies can be overcome using clever AI techniques, including data and model engineering, MLOps for Edge, along with device-specific tuning, deployment, and validation.
- In the future, using tiny AI, enterprise will save millions of dollars in model training costs, gain better environmental credentials, and ease the complexity, privacy, and security challenges of sending huge amounts of data to the cloud.
- Thanks to tiny AI, big enterprise will infuse edge intelligence in customer-centric digital engagements and launch applications that retain value at scale.
AI is already big business but has barely tapped into its potential. The technology is expected to deliver $14 trillion of gross added value to corporations by 2035. 1 This money will come from new business processes, greater synergy between the enterprise and the customer, and the ability to monetize interactions across business ecosystems. With AI, operations are more efficient, delivery is more resilient, and strategy is based on real-time insights.
The bleeding edge of AI includes reinforcement learning in cyber-physical systems, generative networks for drug research, and explainable AI in criminal justice efforts.2, 3 Organizations ranging from the U.S. military to tomato growers in Colombia, which use electric 12-armed robo-weeders to distinguish between ripe tomatoes and other plants, are applying AI to innovate faster, invent new business models, and define new industries.4
As mobile endpoints proliferate, there is a growing need for these AI systems to be more private, responsive, and contextually intelligent. Tiny AI is one of applied AI’s most advanced trends yet. Complex algorithms run on the edge devices themselves and offer real-time predictive ability, even without an internet connection. These advances, however, act as a complement to — rather than a replacement for — current AI systems.
Tech giants and academic researchers are deeply invested in this movement: Most of their recent efforts involve shrinking existing deep learning models without losing their powerful capabilities. The outcome is a plethora of tiny models that pack more computational power into tighter spaces. These tiny systems train and run on a fraction of the energy of their traditional counterparts, and they do all this while keeping information safely out of the hands of would-be intruders by limiting data transfer.5
With tiny AI, models are trained in the cloud and pushed to the edge for real-time problem-solving. Point your mobile phone at a car and the technology can identify external damage and estimate the cost of repairs. Even without an internet connection, Google Recorder algorithms can translate voice to text on a mobile device instantly and identify the difference between a human singing, a pet barking, and a violin playing Bach.6 Health care apps are being developed that can detect heart rate, respiration, and stress — without the need for cloud inference (or prediction) — just by viewing a person’s face. Miniature ARM chips can be embedded in respiratory inhalers to analyze inhalation capacity and the flow of medicine into the lungs.7
Most recent efforts by AI researchers involve shrinking deep learning models without loss of capability
In these examples, the intelligence is aware, data is private, systems are secure, and personal assistance is instant — all far away from the constant hum of interconnected web activity and its vulnerabilities.
Tiny AI: The emergence of the latest trend in applied AI
The popularity of tiny AI has been growing since a 2017 Google white paper described advances in federated learning, confidential computing (the ability to encrypt data while it’s being processed), and the inner workings of Apple’s Siri and Google Now. In 2019, Tensorflow Lite 1.0 — a deep learning framework for on-device problem-solving — was launched and Google’s Tiny ML (machine learning) Community initiative started. In 2020, just three years after tiny AI first made headlines, the NeurIPS annual AI research conference made the term even more popular and relevant. There, researchers offered new techniques in data selection and model compression.8 With 250 billion edge devices and microcontrollers now in circulation, tiny AI is poised to arrive in a meaningful way in 2021.
Most tiny AI applications are currently consumer-driven, but soon, this edge intelligence will transform industry more broadly. The technology will allow devices to work collaboratively on computing-intensive tasks by integrating a large amount of data from smartphones and internet of things (IoT) applications. To get there, inference will need to shift from core cloud providers to distributed edge-cloud local zones, such as factories, plants, and mines.
Moving even closer to the edge, IoT data will be processed by the 3.8 billion smartphones currently in circulation. The final stop on the journey will be a region called the “mist” — decentralized and minimally connected ecosystems of edge devices and sensors with low latency and low capacity embedded in everything from plow forks to thermometers.
The difficulty with tiny AI
As with any burgeoning technology, however, there are naysayers. And some of the doubt is for good reason. Not all AI will work on the edge.
Powering massive models and data-hungry AI algorithms often needs the computational resources provided only by cloud data centers. Cloud machine learning, for instance, is built with massive models, millions of parameters, teraflop processing, and a graphics processing unit (GPU), a tensor processing unit (TPU), and field-programmable gate array (FPGA) hardware. This allows sophisticated algorithms, such as speech analytics, to run effectively without loss of model accuracy or processing speed. On the other hand, tiny ML runs on milliampere per hour batteries that have only megahertz bandwidth to carry data. Edge hardware typically has 100 KB of RAM, which requires accelerators to access data more quickly and efficiently.
For many applications, cloud provides more muscle for important inference tasks. Computer vision and object surveillance are far more powerful in the cloud than on the edge, even with new advances in light detection and ranging technology on edge devices such as the iPhone. For video processing, the cloud provides scene segmentation and multi-camera scene reconstruction; on the edge, you get only basic segmentation and de-noising capabilities.
Further, even when edge devices are heavily involved in inference, the process can be highly inefficient. In the consumer space, federated learning — which allows edge devices to train a global model in the cloud before sending it back to the device for inference — has to happen at night to ensure that smartphone batteries aren’t drained.
Narrowing the AI gap
These weaknesses are offset by the lower latency, lower overhead, and reduced processing costs needed for tiny AI. OpenAI estimates that training costs for natural language models such as GPT-3 in the cloud are estimated at $12 million for just one run.9 Given that multiple iterations are often needed, costs escalate quickly. Further, some tiny AI models actually attain higher accuracy than their cloud equivalents, making their use in inference-sensitive industries, such as finance and health care, very attractive.
The benefits of tiny AI include lower latency, lower overhead, and reduced processing costs
Also, some tiny AI techniques store model weights with fewer bits of data, an approach that can reduce sevenfold the energy needed to train models.10 Other techniques include selecting only the best data before training on edge devices, choosing the right model for the right device, and reducing the number of parameters in the model itself.
Figure 1. Different techniques and approaches used in tiny AI
Source: Infosys
Even though gargantuan AI models still dominate, researchers are pushing forward to ensure tiny AI’s benefits are highlighted and its appeal ripples through boardroom conversations. To determine how best to use tiny AI, organizations need to clearly understand data selection and model compression techniques, along with optimal hardware deployment, as intelligence moves to the edge.
Enterprises will need to understand data selection and model compression techniques, along with optimal hardware deployment for tiny AI
Data selection
With applications such as voice to text, models need to factor in the tone and voice characteristics of the device owner and have a deep understanding of what data is valuable and what isn’t. This is particularly important when training tiny AI models. Ten percent of noisy input can reduce model accuracy by 6%, which can be significant in some use cases. Intelligent learners — classifiers that have control over data selection — are helpful and pick data by something called uncertainty (or diversity) sampling. Here, unlabeled data that are near classification boundaries are chosen based on how close the confidence is to 100%. In some contexts, this AI data selection approach can reduce training data sets from 400 to 270, with a 14% improvement in accuracy.11
Further, our experience at Infosys shows that active learning — a subset of intelligent learning — can reduce by one-third the time needed to label training data.12 Data valuation is also helpful as a way to characterize the usefulness of data via a utility function, such as the Shapley value from game theory.
Tuning and choosing the right edge AI model
Edge devices need small model architectures. Larger models can be tuned to create smaller ones without loss of performance. For instance, VGG-16, a large 500 MB model, has roughly the same accuracy as MobileNet (just 20 MB). To tune the model, AI practitioners need to analyze the number of deep learning layers in the model (and how these layers are arranged). For tiny AI, companies should use more channels and fewer layers and reduce the size of convolution kernels. Also, the right model must be paired with the right hardware. A MobileNet V2 model works well on iPhone XS Max but not very well on Samsung Galaxy Note8 smartphones. To help decide the best fit, architects can use native formats and frameworks that correlate models with performance on different hardware.
Model compression
Most neural networks have too many parameters, which use a great deal of power. However, several techniques can help, including knowledge distillation, network pruning, and quantization.
With knowledge distillation, knowledge from a large model is transferred to a smaller one. While the knowledge capacity of the larger model may be superior, this capacity often is not utilized. Knowledge distillation takes advantage of this discrepancy. This transfer happens through training, wherein the “teacher” trains a “student” and only the most significant layers and weights are utilized (something called “dark knowledge”). In this process, the model architecture of the new network can be significantly different from the original. This technique produces a wider range of probability outputs and increases accuracy on edge cases.
Network pruning assesses the importance of weights in a model and removes those that contribute the least to overall accuracy. The objective here is to create smaller and faster models. With deep learning, this method reduces the number of neurons and connecting circuits, while still providing high accuracy on inference tasks.
Finally, quantization stores the weight of models in quantized units with minimal impact on accuracy. Post-training quantization moves from 32-bit floating point numbers to 8 bits. The models are four times smaller but only 2% less accurate. Also, these models are as much as two to three times faster using the same central processing units.
Taken together, these techniques are ushering in a new age of tiny models that will benefit large enterprises. But for this to happen quickly and at scale, organizations need to focus on privacy and security — not just efficiency and accuracy. Tiny AI models use federated learning, which protects user privacy by enabling AI to scale across edge devices without data transfer. Only the model parameters are sent to the cloud for processing. First-time learning happens on the server, while more specific learning happens locally. When data needs to move to the cloud, differential privacy and confidential computing ensure that AI models work within existing regulatory guidelines, such as Europe’s General Data Protection Regulation. This is particularly important for industries such as finance and health care.
The current state of tiny AI
In 2021 and beyond, companies will need to decide how open or closed their platforms will be and who owns the data that is transferred between parties. The cloud-edge continuum, then, will become ever more important, fueling innovation and enabling democratization. If global models in the cloud conduct all the inference and training, cloud service providers might argue that they too own the data and intelligence generated. Who owns the data and monetization potential from a new Tesla roadster or a new energy conservation system in London?13 However, with new generations of tiny AI, the edge also trains and even collaborates with other devices in the mist. This is already in use with self-driving cars, augmented reality and virtual reality, traffic detection, and smart-city infrastructure. Managing the monetary and reputational consequences of this digital ecosystem play (as the cloud computing fabric bends) will require vigilant policy that doesn’t slow innovation.
Most consumer applications are in generation 4 of tiny AI, while enterprises have not yet passed generation 2
Regardless, companies should act now to ensure they aren’t left behind and lose their share of the profits and success afforded by tiny AI applications. Many companies are already working toward these goals, while others have barely started. Below are the different generations of tiny AI and where they fall along the cloud-edge continuum (Table 1). The more recent the generation, the more likely it is that an enterprise is achieving its desired business outcomes from edge intelligence. In mid-2021, most consumer applications are in generation 4, while most enterprises have not yet passed generation 2.
Table 1. The different generations of tiny AI
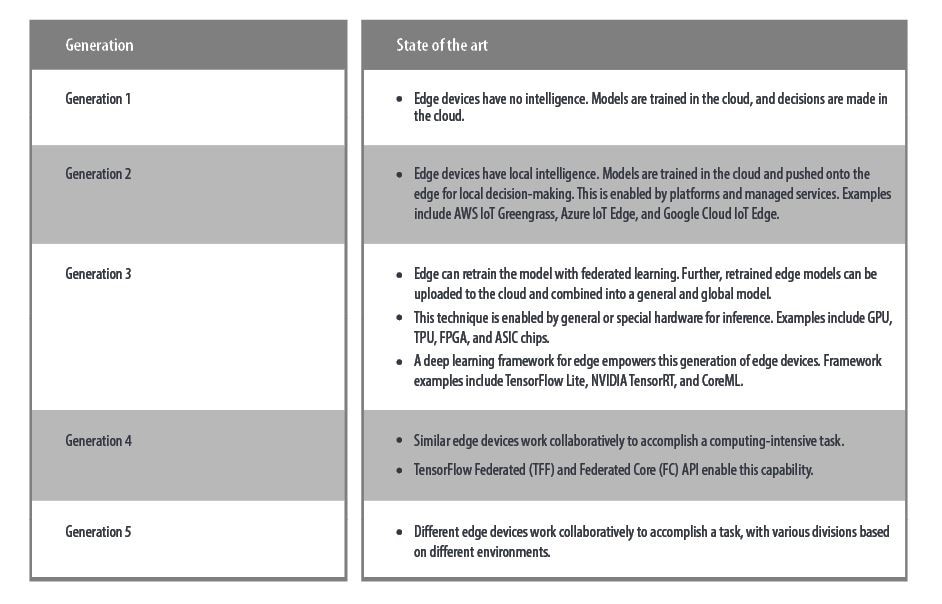
AI’s path to better business outcomes
Recent headlines attest to tiny AI’s broader awareness. Tiny-BERT is a language model that has been widely lauded since its introduction in 2020. Over seven times smaller than the BERT LARGE language model, it achieves nine times the speed, while losing just 4% accuracy.14 It also costs far less to train, so scaling will achieve significant efficiency. Apple iOS 13 on the iPhone uses a technology similar to Tiny BERT for its QuickType keyboard. Simple touches such as this ensure customers return time and again to the Apple ecosystem, forgoing open source apps from more agile upstarts. Apple is also bolstering its tiny AI image recognition capabilities through the purchase of Seattle’s Xnor.ai.15
While Apple’s and Google’s AI efforts have advanced rapidly in the consumer market, most companies are still in generation 2. Much of their AI leans heavily on large cloud providers. However, the relatively small edge AI market is expected to grow at a compound annual growth rate of almost 20% until 2026. Its emergence is expected to coincide with accelerating 5G and electric vehicle innovations, solving the chicken-and-egg problem. Having too few tiny AI solutions increases friction for enterprises, while having too few participants curtails research development.16
And the field will only accelerate with AI skills on the rise in China and the U.S. and the emergence of low-code platforms. These changes will enable enterprises to securely participate in the multiparty ecosystems of the future. “AI was very difficult to do for most companies until now, partly because the number of experts in the field was extremely small,” says Yann LeCun, director of AI research at Facebook. “This shortage is easing, as even young graduates now have knowledge of AI techniques. There are also tools and platforms being built for people who are not yet experts to get started on developing AI applications.”17
As cloud grows to imperial dimensions, edge AI will be the suave aristocrat working behind the scenes. Using tiny AI, enterprises stand to save millions of dollars in AI model training costs, gain better environmental credentials, and ease the complexity, privacy, and security challenges of sending huge amounts of data to the cloud. Big enterprise will infuse edge intelligence in customer-centric digital engagements and launch applications that retain value at scale. These smart machines, powered by tiny AI chips, will also help expand markets. Firms will be able to participate in a common marketplace that brings together industries across the health, manufacturing, construction, logistics, agriculture, and energy domains.
Perhaps this new dawn will make large enterprises more people-centric. Bureaucracy can give way to a world where experiences are more enjoyable and perceptive (with users’ needs ascertained ahead of time). Because of this, productivity flourishes, and business executives use fifth-generation tiny AI tools to turn software into gold. “Hope is passion for what is possible,” said Soren Kierkegaard, the Danish philosopher. A world where those Colombian tomato growers significantly increase seasonal yield is certainly a hopeful one, even while their digital assistant comes alive at the sounds of birds passionately chirping in the bush.
References
- Implementing AI in business – challenges and resolutions, Harry Keir Hughes and Isaac LaBauve, Nov 2019, Infosys Knowledge Institute
- 6 unique GANs use cases, July 2019, Open Data Science — Medium
- A case for explainable AI & Machine Learning, 2018, Katarina Athens-Miller, Anna Olecka and Jason Otte, KDnuggets
- How AI is taking over our gadgets, Christopher Mims, June 2021, Wall Street Journal
- Tiny AI, Karen Hao, Apr 2020, MIT Technology Review
- Google Recorder: The smartest recorder yet, Google
- Bringing AI to the device: Edge AI chips come into their own, Duncan Stewart, Mark Casey, Jeff Loucks, and Craig Wigginton, Dec 2019, Deloitte Insights
- Tiny four-bit computers are now all you need to train AI, Karen Hao, Dec 2020, MIT Technology Review
- OpenAI’s massive GPT-3 model is impressive, but size isn’t everything, Kyle Wiggers, June 2020, Venture Beat
- Tiny four-bit computers are now all you need to train AI
- Scaling AI: Data over models, Rajeshwari Ganesan, Sivan Veera, and Harry Keir Hughes, May 2021, Infosys Knowledge Institute
- Scaling AI: Data Over Models
- Training at the Network Edge with AI: 3 Key Benefits, Yasser Khan, May 2020, RT Insights
- TinyBERT – size does matter, but how you train it can be more important, Viktor Karlsson, Apr 2020, dair.ai
- Global edge AI market 2021-2026 – rising demand for innovative & advanced vehicles, Apr 2021, Business Wire
- Global edge AI market 2021-2026 – rising demand for innovative & advanced vehicles
- Artificial intelligence in the real world: The business case takes shape, 2016, The Economist Intelligence Unit