The human touch in artificial intelligence-aided trading
Technology has dramatically changed capital markets, a space that was historically defined by its reliance on humans and their voices to trade and run its daily business. Nearly 80% of daily volumes in the U.S.1 are now traded electronically using algorithms, rather than on the open outcry trading floors that were prevalent until the end of the last century.
It is not only operational efficiency that has driven this adoption, unlike in other industries. Capital market players have deployed algorithms and AI capabilities for competitive advantage, enabling more complex products and fueling higher trading volumes.
However, the reliance on computers to make investment decisions, often at high speed, can create catastrophic risks. In this new era of AI adoption, the time has now come to consider how humans can be reintroduced into the process to act as safety valves.
Algorithms and AI in capital markets
Banks have been using computers to make decisions on trades for decades. In their most basic form, algorithms are used to create investment strategies across a complex portfolio, and then used to execute that strategy.
Execution effectively automates the breaking down of a large trade into smaller lots to allow the trade to be executed at the right price over time.
Decisions are still made by human traders, but these decisions are informed by, and then executed through, algorithms.
The most sophisticated algorithms drive high-frequency trading, which does not involve any human intervention. Competitive advantage is gained purely through being the fastest to respond to market movements and to capitalize on them — both through having the lowest latency algorithms and being physically nearest to the exchange’s own computers.
In recent years, trading technologists have started to experiment with artificial intelligence. This takes decision-making beyond algorithms designed for speed, strategy and execution and into the world of cognitive decision-making. AI uses neural networks that scan multiple data sources and effectively replace the human.
The risks of algorithmic trading
Algorithmic trading does not have an untarnished reputation. While it works most of the time, when it goes wrong, it can be disastrous.
In 2012, Knight Capital, a market maker with over 17% of market share on the New York Stock Exchange, was an early advocate of algorithmic trading. However, in August of that year, the firm lost over $440 million in 45 minutes when its new algorithm went live. The algorithm was flawed and triggered one of the worst collapses in trading history. Stock prices of hundreds of companies went into a downward spiral.2
This wasn’t the first example of a problem with algorithmic trading. The “Flash Crash” of 2010 began when Waddell & Reed, a mutual fund house, initiated an order to sell $4.1 billion worth of S&P 500 futures contracts through their algorithmic trading system.3 The order sucked the liquidity from the market and caused the Dow Jones Industrial Average to collapse 9%, depleting $862 billion4 of investor wealth and sending stock prices of market leaders, including Apple, Accenture, GE and P&G, significantly lower.
This doesn’t change the fact that algorithmic trading has played a big role in helping trading volumes to increase by 10 times5 since the early 1990s. For the most part, it has created a net benefit through lower trade cost, a higher probability of trade execution, a tighter bid-ask spread and increased market liquidity. Banks are now taking algorithmic trading further and experimenting with AI.
Artificial intelligence for competitive edge
In the past decade, banks and hedge funds have poured money into developing AI trading platforms that can be intuitive and make strategic decisions.
The expectation is that AI will provide a further competitive edge over rivals and enable the creation of new and even more complex products.
In 2014, Goldman Sachs led a $15 million funding round for an AI platform called Kensho.6 The platform uses machine learning to scan through millions of market data points, looking for reasonable correlations and arbitrage opportunities. For example, during news of the Syrian civil war, Kensho offered insights to traders on commodities and currencies within minutes that would have otherwise taken days to gather.
Founded in 2011, Aidyia deploys AI to crunch large volumes of data, identify and predict patterns invisible to the naked eye, and evolve its trading strategies. The fintech’s deep learning and neural network reads and interprets sentiments from news articles and social media, while its natural language processor analyzes and processes data from various languages. All this is done without human intervention.
The jury is still out on AI performance
Despite the investment and focus on AI, the techniques are still in their infancy, and there have been several high profile AI failures as well. Babak Hodjat, who helped build Siri, and Antoine Blondeau spent nearly 10 years developing an AI system that rifled through trillions of datasets and used techniques of evolutionary computation to create a set of “super traders.” Blondeau believed that if AI could be taught to drive autonomously, beat humans at strategy games and understand multiple languages, it could surely trade. Sentient Investment Management’s AI-managed fund began accepting private investors in 2016. Two years later the hedge fund was liquidated. With assets under management of about $100 million, it made 4% in 2017 and nothing in 2018.7
On February 14, 2018, there was no love lost between Hong Kong real estate tycoon Samathur Li Kin-kan and Tyndaris Investments, whose robo-powered hedge fund cost Li $20 million that day. Samathur, who handed Tyndaris Investments $2.5 billion to manage, bought into the idea of K1, a supercomputer that would crawl through news and social media in real–time, sense investor sentiments and predict U.S. stock futures. Li intended to double his returns. However, things didn’t go as planned and he began to lose money from the minute he invested. He is now suing the firm for $23 million.8
Even when things do work, it seems that strategies formulated by AI–managed funds do not always outperform benchmark indices. For instance, the Eurekahedge AI Hedge Fund Index that employs AI and machine learning (ML) grew at a 5-year CAGR of 8.3% between 2014 and 2019 (Figure 1). While this was better than the 5-year CAGR of a Hedge Fund Index (global, equal-weighted index of over 1,400 single-manager funds), it underperformed the 10.9% rise of the S&P 500 Index.
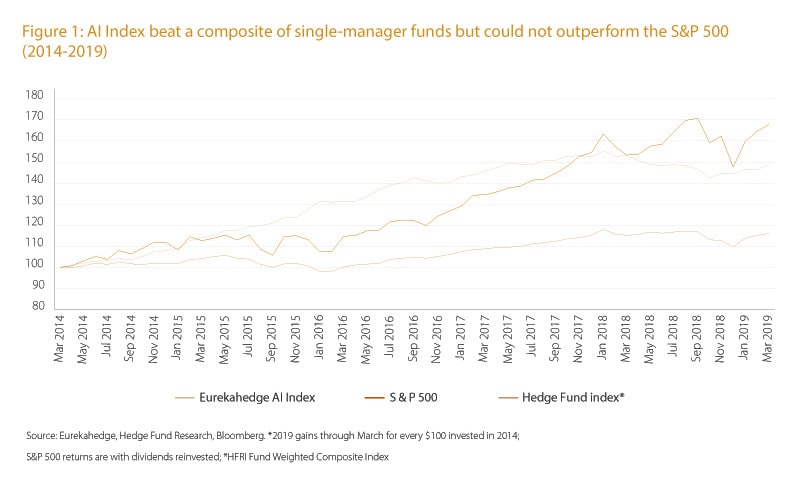
Interpretability is the next big challenge
Despite the teething issues, there is no doubt that AI will increasingly influence, and eventually manage, a significant proportion of trading because it can manage higher volumes and more complex decisions at speed. But it is unclear that AI will be better than humans at understanding structural risks such as the U.S. mortgage market crisis over 10 years ago or at avoiding flash crashes.
We believe humans will remain instrumental in providing a guiding hand over AI-driven trading. In order to do this, the complex and fast-paced decisions need to be interpreted and understood. That won’t be easy.
As AI gets better, increasingly through deep learning techniques, understanding the lineage of a computer-made decision will only become more complex.
As Stanley Druckenmiller, former chairman of hedge fund Duquesne Capital, puts it, “These algos have taken all the rhythm out of the market and have become extremely confusing to me.”9
Ironically, the challenge that traders and engineers face in explaining a computer-generated strategy is also what stops many AI- and ML-based algorithms from seeing the light of day. The challenge in explaining how an algorithm works is sometimes a big drawback to the implementation of AI-based initiatives.
From a bank’s perspective, solving the “interpretability” conundrum would boost management’s confidence in dealing with regulators, investors and decision-makers to allocate budgets to AI projects and would help traders make effective use of strategies. More importantly, it would help avoid, or at least minimize, catastrophic losses. Humans are not central to creating strategies anymore, but they play a more vital role in interpreting AI decisions and acting as guiding hands. The future lies in humans coexisting with AI while at the same time acting as a safety valve.
Finding the skills to interpret
It will be hard to find and develop the skills required to interpret and look out for the warning signs of AI failures. Already, banks are pursuing individuals with dual skills — traders who can code or coders who can trade — rather than focusing on one-dimensional skill sets. This has spurred a demand for people from STEM (science, technology, engineering and mathematics) fields, including Ph.D. physicists, quantum-computing Ph.D.s, statisticians or data scientists. Of the 36,000 employees at Goldman Sachs, nearly 25% are engineers.10
A growing number of new hires will need to be trained not just in designing AI-based systems and strategies, but also in understanding and managing AI systems that will increasingly be able to design and build themselves. The future could be less about human traders and more about AI caretakers, doctors or police. The ability to detect and manage unexpected threats from AI systems must be developed in order for AI to be truly embraced by the capital markets.
References
- “Sell-offs could be down to machines that control 80% of the US stock market, fund manager says,” CNBC, December 2018
- “The Rise and Fall of Knight Capital — Buy High, Sell Low. Rinse and Repeat.” Medium, August 2018
- “The 2010 'flash crash': how it unfolded,” Guardian, April 2015
- “High-Frequency Follies,” Forbes, January 2012
- “Secular Stock Volume Decline Not Unprecedented,” The Lyons Share, July 2014,
- “Wall Street Tech Spree: With Kensho Acquisition S&P Global Makes Largest A.I. Deal In History,” Forbes, March 2018
- “AI Hedge Fund Is Said to Liquidate After Less Than Two Years,” Bloomberg Quint, September 2018
- “Captain Magic’ Clashes With Tycoon Over $5 Billion Hedge Fund,” Bloomberg, March 2019
- “Stanley Druckenmiller: Algos Have Taken The Rhythm Out Of The Market,”, The Acquirer’s Multiple, October 2018
- “Computer engineers now make up a quarter of Goldman Sachs’ workforce,” CNBC, April 2018