To get the best from data, every large corporate needs a strategy. But why is it so difficult to formulate one, and in an ideal world, what would one look like?
Be more competitive with data
In 2009, Google tested 41 different shades of blue for its advertising links. This initiative was spearheaded by former executive Marissa Meyer. Bringing customer feedback and data analysis together, Google found just the right color — at the same time as it lost a few bored and disgruntled marketing executives. Finding just the right shade increased Google ad revenues by $200 million that year as more people clicked links than before1.
Netflix collects data from 151 million subscribers. Advanced analytics are used to understand customer behavior and buying patterns. Netflix recommendations have seen the media giant’s value soar to $164 billion, with customer retention at 93%2.
Walmart collects 2.5 petabytes of unstructured data from 1 million customers every hour2. It uses optimization and simulation algorithms to price its products in real time, factoring in insights from trend, weather and economic data. This data — what customers buy online versus in store, what is trending on Twitter or how local weather deviations affect buying patterns — has seen the retail behemoth increase sales from $309 billion in 2006 to $514 billion in 2019.
It is clear that good use of data can make firms more competitive, help them save money, improve business processes, increase customer centricity and develop ground-breaking marketing strategies. Additionally, proficient use of data enhances operational efficiency, mitigates risks and generates new revenue models. It’s no surprise that corporates are rushing to embrace data analytics solutions.
By 2023, the advanced analytics market will be worth $40.6 billion, with a compound annual growth rate of 29.7%4. However, according to Infosys analysis, over half of large corporates are yet to implement a robust data strategy, with many running various proofs of concept and pilots without an end-to-end blueprint in place5.
So why are firms falling short? Why is it so hard to formulate a strategy?
The difficulty with data analytics
The three biggest challenges we see within businesses successfully adopting data analytics techniques are a lack of a data-driven culture, lack of analytical and technical skills, and lack of experience in applying analytics to business issues.
Changing culture is difficult6. It requires an ongoing investment of time, effort and money, with regular pit-stops to track progress and instill new ways of working. Often, when firms invest so much effort and see little reward, they give up and return to the status quo. These firms also lack top-down decision-making and the empathy needed to bring leaders and fast-moving teams together effectively. They get caught in long cycles of decision-making and waterfall methodologies that see products and services enter the market too late on the trend curve.
Lack of a data-driven culture, skills in analytics and experience are dominant challenges faced in big business
Data itself is also often difficult to use. It’s often unstructured (think video, images and diverse text files), trapped in outdated databases, while processing it requires optimal use of cloud technologies7. Legacy systems, for their part, do not and cannot take advantage of the features native to advanced technologies such as machine learning and advanced analytics.
Experience is also an issue. As we wrote in the report Infosys Digital Radar 2019: Barriers and Accelerators for Digital Transformation, firms that lag in their data initiatives find it difficult to become “visionary” organizations — those that understand that future success depends on learning to think and act like digital natives. They often implement digital technologies without true appreciation for the inherent challenges posed by data — such as the need to experiment quickly, employ sufficient budget for each initiative and work across siloed business units. Many lose heart and give up before they even really begin.
Four steps to a data strategy
1. Build a data-driven culture
First things first — firms must build a data-driven culture. New digital technologies won’t take root without a data initiative that extends across the firm.
To do this, firms must employ data scientists who understand the business and how data may be used to improve finances. In an ideal scenario, a mining company would employ data experts with mining experience and an appreciation for how the value of data changes at different stages in the mining life cycle. However, this ideal situation is rarely found in practice. Expert data scientists are in short supply, with demand for workers with specialist data skills more than tripling over the past five years8.
In our POV Implementing AI in Business — Challenges and Resolutions, we recommended building a new operating model wherein data scientists are at the top of a pyramid, with layman “citizen scientists” and business executives with some knowledge of data models and implementation challenges forming the base (Figure 1).
Figure 1. The data analytics skills pyramid
Those at the top — data scientists with specialized expertise — should be “street smart,” according to Jitendra Raisinghani, a principal architect at Infosys, who has led a data migration to Google Cloud. “These people know how object-oriented things work, can pick up new technologies easily and are used to working with agile,” he says. They are also able to share their knowledge with those at every level of the skills pyramid, effectively changing how everyone in the business makes decisions, from small, tactical resolutions to mission-critical strategic changes.
To assist in this, those at the bottom of the pyramid can plug data into standardized templates for generating insights9 while others can use knowledge sharing (or “collaborative ideation”) platforms to socialize data intelligence up and down the data hierarchy. These technologies (often bought as a service from vendors such as SAS and Oracle) connect humans and data in a fast, self-service manner that outperforms traditional metadata approaches10.
Business executives can thus become enmeshed in a data-driven culture where, according to Craig Le Clair, principal analyst at Forrester Research, “technology turns every employee into a programmer”11. For instance, one British multinational oil and gas company Infosys worked with has developed a data marketplace for internal sharing and consumption of their data among different business units. This marketplace has increased data discovery, improved data security and compliance, and increased collaboration, with the whole company working on projects that deliver significant ROI.
Figure 2. Data specialists, executive sponsors, citizen data scientists/advocates and business/functional experts make up the skills pyramid
2. Meaningful executive leadership
Second, this data-driven operating model should be designed with more than just executive buy-in and support. It should make leaders fully embrace data-led decisions and set the culture through their own example. This is critical. Brent Dykes, senior director of data strategy at Domo, a cloud-based business intelligence platform, says, “If the desire to be data driven begins at the top, it frequently cascades down throughout an entire team or company.”12
Rather than relying on rock-star data scientists (who come at a huge cost), businesses should train all their executives in the whys and wherefores of data analytics. These executives must lead by example and demonstrate a servant-leader mentality, where those at the base of the skills pyramid can speak up and make their voices heard.
Much of data analytics work is experimental. Therefore, leaders must be prepared to be challenged and take corrective action when their decisions don’t deliver on strategic business. They must show that they, too, use data on a regular basis; once employees see their leadership diving into the more difficult aspects of data analysis and representation, they will take courage and start to ask more questions.
Even more important, leaders should visibly use data analysis to make key decisions that affect the whole company. There is no better boon to a data strategy and a well-functioning skills pyramid than an overarching understanding that what data scientists and their teams are doing actually matters in the business cosmos. For this to occur, both business and data executives must sit down together, communicate and promote data-driven wins, with business executives setting the agenda in most cases.
These meetings should also serve to develop action plans based on data-driven results, which in turn allow the firm to pivot quickly and ensure a defective product or service doesn’t make it out the door. For more mature firms, these meetings are a good time to think not only about tactical wins but also about more strategic and goal-oriented approaches to add to the bottom line.
3. Find some quick wins that inspire confidence in data
Quick-win projects remove much of the lethargy that inexperienced firms suffer on their journey to data-driven excellence. These projects also increase confidence in the data and tools used. Dr. N.R. Srinivasa Raghavan, chief data scientist at Infosys, points out that firms stand to gain a lot from having a unified view of data, which often comes by approaching a difficult (though explainable) challenge head on. In turn, these projects inspire an agile way of working and promote collaboration up and down the skills pyramid.
For firms in sectors such as financial services or retail that are sitting on a lot of historical data, using techniques like natural language processing, text analysis and data mining can uncover a 360-degree view of what’s going on behind the scenes. Customer segmentation is a great place to start here, with insights into who bought what, when, where and how delivering immediate ROI so that the business can take further actions, such as new pricing and marketing strategies.
There is nothing like seeing a business strategy deliver quick results, whether improved operational performance, higher levels of customer centricity or new-found profits in nontraditional markets. These quick wins will in turn increase the reputation and the attractiveness of the organization to those in the business who remain skeptical of the benefits.
4. Augment human intelligence
Adopting a data-driven culture can be scary. Good analytic practices can expose inefficiencies and threaten incumbent teams, executives or functions within a business. Moreover, as data analytics becomes established, it forms the foundation for more automation and artificial intelligence applications, further threatening the traditional roles of employees.
Therefore, throughout the formation of a data strategy, one key concept must remain at the forefront: Human intelligence is being freed up to focus on softer and more creative skills; it is not being replaced by analytics and the artificial intelligence solutions that it will inevitably support.
The data backs this up. Recent research by Infosys Knowledge Institute found that businesses value soft skills such as teamwork, leadership and communication significantly more than technical skills such as analytics, automation or artificial intelligence. Skills such as creativity, critical thinking and adaptability also are valued as much as, if not more than, these harder technical skills13.
A good data strategy is one where human intelligence is freed up to focus on softer and more creative skills
Adopting a strong data analytics strategy is an opportunity to release the pent-up creative, collaborative and critical capacity within organizations. It enables this more human intelligence to focus on asking the right questions of analytics tools; interpreting the results into effective product, service and customer strategies; and engaging in more valuable employee and customer experience skills.
For example, one fashion and sports apparel brand Infosys worked with found that they could use machine learning to gain a greater understanding of emerging trends in their “couture” vertical. They created a new data-driven sales department that developed pattern-matching algorithms to find the size, position and color of specific logos that correlated to the greatest number of sales over a given period. This would not have worked with algorithms alone, but relied on human understanding of the product and its values.
Humans are still the most valuable asset for businesses, and this fact needs to be established early on within an organization’s data analytics transformation so as to not alienate or threaten the colleagues that a business so desperately needs to make any holistic strategy stick.
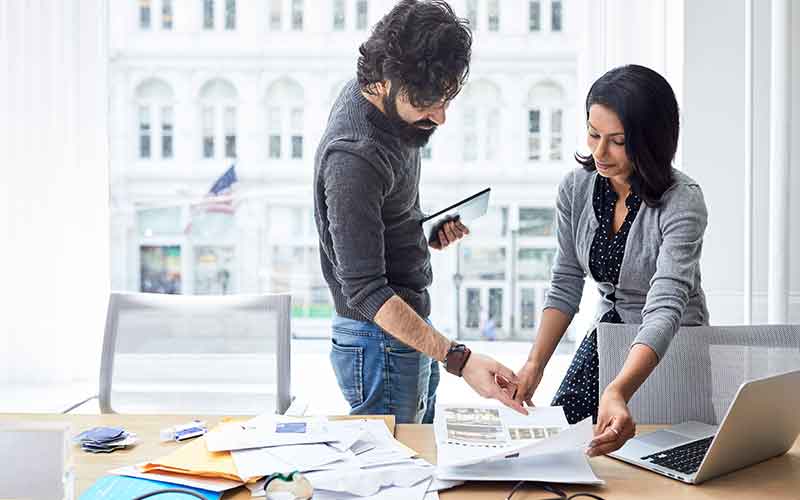
Building a ‘Live Enterprise’
The end goal of this data strategy should be what Infosys calls a “Live Enterprise”14 — a completely knowledge- and data-driven organization, with agility built into its DNA so that it can quickly sense changing business needs and continuously evolve in response. To get there, the operating model must have both the employee and the customer at its center, and a strong business core must be built up where the network of partners, suppliers and customers talk to each other in real time, intelligently and at the speed of data transmission.
However, to achieve this level of sentience, careful attention must be given to how data is used, stored and acted upon. Employee, customer and partner privacy must be paramount. Also, business leaders must make some of the more challenging decisions for the enterprise with balance in mind. The innate understanding of uncertainty and ambiguity that makes humans unique will remain front and center, with clever algorithms suggesting new audiences, new market opportunities and effective customer engagement strategies. For this to occur, we recommend the business has as close to a flat hierarchy as possible.
The goal of this strategy is the enterprise “as a platform for innovation,” adapting quickly and finding new ways of making a profit while remaining transparent, sustainable, ethical and scalable. Once firms reach this holy grail, they can start thinking about monetizing their data (much like Google, Amazon and Infosys) and giving customers what they want, how and when they want it.
References
- Creating A Data-Driven Culture: Why Leading By Example Is Essential.
- How Netflix used big data and analytics to generate billions.
- How Big Data Analysis helped increase Walmarts Sales turnover?
- Global Big Data Analytics Market, Forecast to 2023, Frost & Sullivan.
- Endless Possibilities With Data, Survey Report, Infosys.
- The Behavioral Change Journey, IKI.
- Implementing AI in Business — Challenges and Resolutions, IKI.
- Growing demand for data science leaves Britain vulnerable to skills shortages.
- Yes, You Too Can Be an AI Expert.
- The Sentient Enterprise: The Evolution of Business Decision Making, Wiley.
- See Reference 9, above.
- Creating A Data-Driven Culture: Why Leading By Example Is Essential
- Infosys Talent Radar 2019: How the Best Companies get the Skills They Need to Thrive in the Digital Era
- Infosys Live Enterprise — A Continuously Evolving and Learning Organization