Insights
- Many firms initiate their AI journey with a closed-access large language model, especially given their capabilities in text-generation, and then progress to open-access models.
- Open models offer customization and transparency. In contrast, extensive training on vast public data sets strengthens closed models. But they lack transparency and disclose little about their training and architecture.
- Regardless of which model is chosen, new entrants to generative AI need to navigate both app- and enterprise-level risks and develop a holistic responsible by design toolkit to implement the technology at scale.
Businesses bet big in generative AI to explore its operational potential. This brings concerns too. Companies currently debate over whether to use open- or closed-access large language models, the technology behind applications such as ChatGPT and other retrieval augmented generation apps like Perplexity AI.
Open-access development decentralizes model influence as a group of stakeholders shape AI systems based on their distinct needs and values (e.g., BigScience’s Bloom). Closed-access systems, like Google’s LaMDA or OpenAI’s ChatGPT, resist outsiders but lack external research or auditability.
There are advantages and disadvantages to both. Open models offer customization and transparency. Firms utilize them for tasks such as custom coding assistance or for fine-tuning their domain assistants. However, these models may not be available for commercial purpose, and, as in the case of Meta’s Galactica, may be shut down after unreliable performance. In contrast, extensive training on vast public data sets strengthens closed models. But they lack transparency and disclose little about their training and architecture. Both models struggle with unsophisticated prompts.
Many firms we converse with initiate their journey with a closed model, especially given their capabilities in text-generation, and then progress to open models. This duality drives Infosys to offer diverse generative AI models (150+ under Infosys Topaz).
New entrants to generative AI need to navigate both app- and enterprise-level risks and develop a holistic responsible by design toolkit to implement the technology at scale.
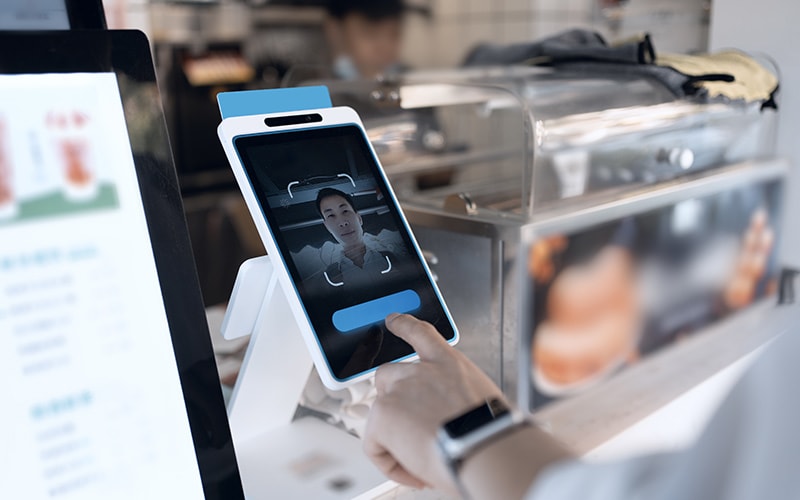
Are closed models better?
Firms beginning to incubate these capabilities prefer closed models consumed as a service. They are easy to implement, provide faster time-to-market, and require lower upfront costs. In contrast, open models require specialized capabilities around talent, data, infrastructure, and tooling, and involve licensing restrictions. Certain open models are only available for research use.
Firms beginning to incubate generative AI capabilities prefer closed models consumed as a service.
Enterprises use closed models even without fine-tuning, aided by retrieval augmented methods (which improves AI trust and quality) such as for semantic search. They benefit from an established cloud ecosystem that offers security and privacy controls.
Moreover, app developers actively assist enterprises to build and rollout products based on closed models. Our flagship AI research report Tech Navigator: The AI-first organization advocates firms to invest in prompt engineering first, before utilizing traditional machine learning skills. Prompt engineers teach foundation models to reason accurately and understand the interplay between foundation models, external systems, data pipelines, and user workflows.
Figure 1. Open and closed models’ comparison
Key attributes | Open | Closed |
---|---|---|
Examples | Falcon, BLOOM, Whisper, etc. | OpenAI GPT 4.0, Anthropic, etc. |
Pricing | Free | Pay-per-use |
Skillsets | Programming (high), ML (high) | Programming (high) |
Time to build use cases | High | Low |
Infrastructure | High | Minimum |
Training data, model weights, and LLM architecture | Open | Closed |
License | Noncommercial use OR Apache 2.0 | Only API call |
Type of AI roadmap | Narrow AI | AGI |
Use cases | Enterprise-driven | Consumer-driven |
Trained on | Fine-tuned or curation with private data | Massive amounts of public data |
Purpose | Task specific or domain specific | General purpose |
Internal tools (e.g., MLOps) | Very important | Not important |
Risks | Low | High |
Source: Infosys
Navigate risky territory of closed models
Figure 2 shows four key risks with closed models, applicable also to open models at varying degrees.
Figure 2. App and enterprise level risks of closed models
Source: Infosys
Data risk amplifies in closed models. For instance, GitHub Copilot, a closed model, underwent training on code that could potentially have licensing issues. Firms, including big news providers, rewrite licenses and contractual agreements around their content, and update websites to stop generative AI scraping their data. Data poisoning, where content is spiked with deliberately malicious or misleading information, threatens web-trained models. Some latest closed models such as GPT 4 are able to memorize, but not to learn. To benchmark GPT-4’s coding ability, OpenAI used problems from Codeforces, a coding competition website. The model solved all pre-2021 problems but cracked none beyond that. It suggests the model memorized, not learned, solutions from its September 2021 training cut-off.
Italy earlier banned ChatGPT’s launch due to personal data concerns and inadequate youth content safeguards. The model returned for Italians after amendments required by Italy’s data protection authority.
Firms often lack insight into a model's training data, biases, and issues. As large enterprises embrace closed models, they can't shape the foundation model's core limitations.
As large enterprises embrace closed models, they struggle to shape the foundation model's core limitations
In model risk, foundation models struggle to comprehend their creations; chatbots built on them often produce completely arbitrary and incorrect responses (known as hallucination). In their most primitive form, they are next-word predictors. Computational linguist Emily M. Bender refers to them as stochastic parrots. Spreading misinformation, disinformation, and falsehoods stand among the worst offences. Infosys experts say generative models maintain truthfulness only 25% of the time; the rise of deepfakes blurs the line between truth and faux.
In prompt injection, attackers use carefully crafted prompts to make the foundation model ignore safety guardrails such as around creating violent or malicious content.
User risk involves unintentional spread of misinformation and harmful content by users. For instance, some might mistake AI-generated hallucinations for accurate information.
These four risks further split into app-level risks, where outputs suffer from poor privacy, safety, security, availability, and reliability: or enterprise-level risks that concern IP, regulatory offenses, and reputational defamation hazards.
Both closed and open models have risks. Firms should use external control systems and risk frameworks when buying off-the-shelf commercial models.
Better external control methods
Firms should address app-level risks through external controls such as human oversight to optimize outputs, knowledge injection, and enterprise-level cloud controls. For instance, access ChatGPT API through Azure OpenAI Service, a cloud container. The service encrypts data in transit, filters content, removes personal data, and prevents prompt injections. If a user types a prompt into Azure OpenAI service (requesting car repair after an accident and stating personal details), the cloud system will encrypt all personal data that gives away the driver’s personal identity. This level of security lacks when typing prompts directly in the API-level chat window.
This cloud container checks compliance, filters hallucinations, and flags potential copyright violations and sensitive data exposure. It stores all prompts as immutable assets, which means better explainability and transparency. Privacy amplifies as any data sent through the API from the customer only has a short shelf-life and destroys thereafter.
A responsible AI toolkit
Beyond tech, companies require a responsible AI toolkit, as WHO issued in 2021.
Here, accountability, fairness, data privacy (and selection), transparency, explainability, and value and purpose alignment hold significant importance. Accountability means consumer education on potential risks, responsibilities, and sensitivities.
Firms require ethical frameworks to handle training data and reduce copyright and consent issues. If transparency and explainability are embedded in these models from the start, the techniques discussed above become less significant. Foundation models will then show the logic behind their work.
The development of truthfulness indexes for generative AI systems reflects growing awareness of ethical AI. Truthful QA ensures AI models generate accurate and reliable information, while IBM’s AI 360 Toolkit offers industry-grade tools to assess and improve fairness, explainability, and robustness of AI systems. Microsoft, Google, Meta and Amazon have also released their indexes.
What’s next
While closed models gain traction, firms must reduce inherent risks. They use retrieval augmented generation, chain of thought (CoT)/tree of thoughts (ToT) prompting, and other advanced prompt engineering to do so. Some scale cautiously with human-in-the-loop and unified business-IT ethics discussions.
Some firms scale generative AI cautiously, with human-in-the-loop and unified business-IT ethics discussions
Some at Infosys prefer narrow transformers trained on company data. Open models like Bloom, Llama, and Alpaca offer insights into their inner workings. But even with open models, we can’t control everything; fine-tuning remains crucial, and it doesn’t remove pre-existing weaknesses.
Bigger and closed models don’t always mean better; yet, currently, they are. Our trial of smaller and open models revealed limitations in knowledge-intensive tasks. Foundation models’ control issues require a closer look at what technology like ChatGPT does well, and integration of control technology and ethics toolkits ensure widespread AI deployment.