Webinar: Learn How Tyson Foods’ Appetite for Data is Customer-Driven
Many CPG companies are investing heavily in advanced analytics – which is no surprise given that data and analytics at scale can generate a 5-10% uplift in revenue.
However, implementation at scale is where the problem lies. It is estimated that about 70% of the typical issues that prevent companies from unlocking their full advanced analytics potential are due to a missing link between business and data science.
This is why the insights provided by Lee Slezak of Tyson Foods is so compelling. As VP of IT Architecture, Emerging Technology & Analytics, he is leading a digital transformation at a major CPG player – and shares his insights with us on how to make a success of AI and advanced analytics in a CPG environment.
Insight #1: How to communicate the power of AI internally
When I joined no one knew what AI was or what it could do. We did lots of roadshows early on – demonstrating possibilities. It’s important to connect the dots for people, showing how it can be used in their everyday lives. But then there’s a flywheel effect. We did one computer vision project to replace error-prone manual processes. That was really successful, and they saw it had a business benefit – then suddenly everyone wanted to talk to us.
Insight #2: prioritization problems
So how do you prioritize requests? In the early days it was who had the biggest budgets and who shouted loudest. But we then put together a proper governance program where there is a steering committee who prioritize more rigorously. We decide whether it is feasible – and more importantly whether it’s scalable. It’s only then that we know if we’re getting the biggest bang for our buck.
Insight #3: focus on efficiency first
We started by driving efficiencies and cost savings instead of working on revenue generation ideas because they’re the easiest ones to justify and sell across the organization. For us it might be safety solutions or quality and grading solutions for products at first. As we move forward, we started to focus on areas like supply forecasting, demand forecasting, demand sensing — those are key areas where we're investing.
“They saw it had a business benefit – then suddenly everyone wanted to talk to us.”
Insight #4: how to solve the skill shortage
It’s really hard to find the right talent. Partnering is of course a great way to find the skills, but AI and analytics are so strategic we want to keep that knowledge in the organization. We have a few different ways of retaining our talent: we make sure that we have the best capabilities and tools for our team members to work with, and we spend a lot of time recognizing the work that they're doing. We give them the visibility to grow in their careers, as well as to add value for the company. We aim to bring people in early in their careers and help them grow.
Insight #5: create a data lake – not a data swamp
It’s easy to focus on the engineering aspects of bringing data into a lake, but don’t ignore the part about organizing it for democratization and consumption or you're probably going to be creating a data swamp. We put our energy into the modeling of that data. We focus on the intersection of the technologists with the business subject matter experts to understand what data needs to be grouped together so that we can get the most value out of it. It’s hard work and there is no real answer but persistence and hard work but paying attention to master data and making it trustworthy is essential.
“Paying attention to master data and making it trustworthy is essential”
Insight #6: start small, move fast, think big
Whether you're looking at AI or any technology initiative, start small. Start with a well-defined scope but move fast. The half-life of these initiatives, especially without full organizational support or acceptance, is short. But then you need to think big and show how that small piece of scope proves what is possible when working at scale. Then move as fast as you can to get it socialized, scaled, and deployed.
Partnering can help to achieve scale quickly, particularly in terms of supporting it on an ongoing basis. We partner with cloud companies and experts like Infosys who bring in experience in different areas. I see it as a ‘by-all-means-necessary’ approach: I don’t just set out to hire the best team. If we bring in smart people and grow them, but at the same time partner with the best people on the planet, we’ll get the best possible results.
“If we bring in smart people and grow them, but at the same time partner with the best people on the planet, we’ll get the best possible results.”
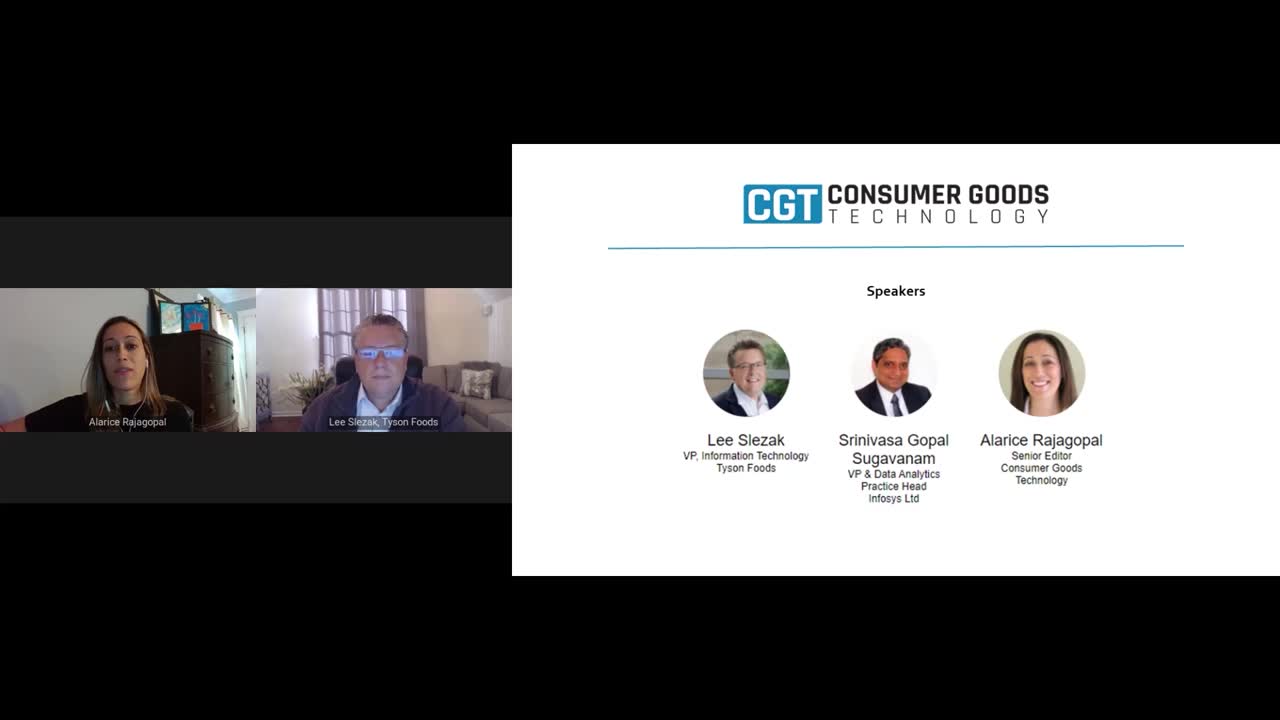